September 2, 2023
Splendid Edition
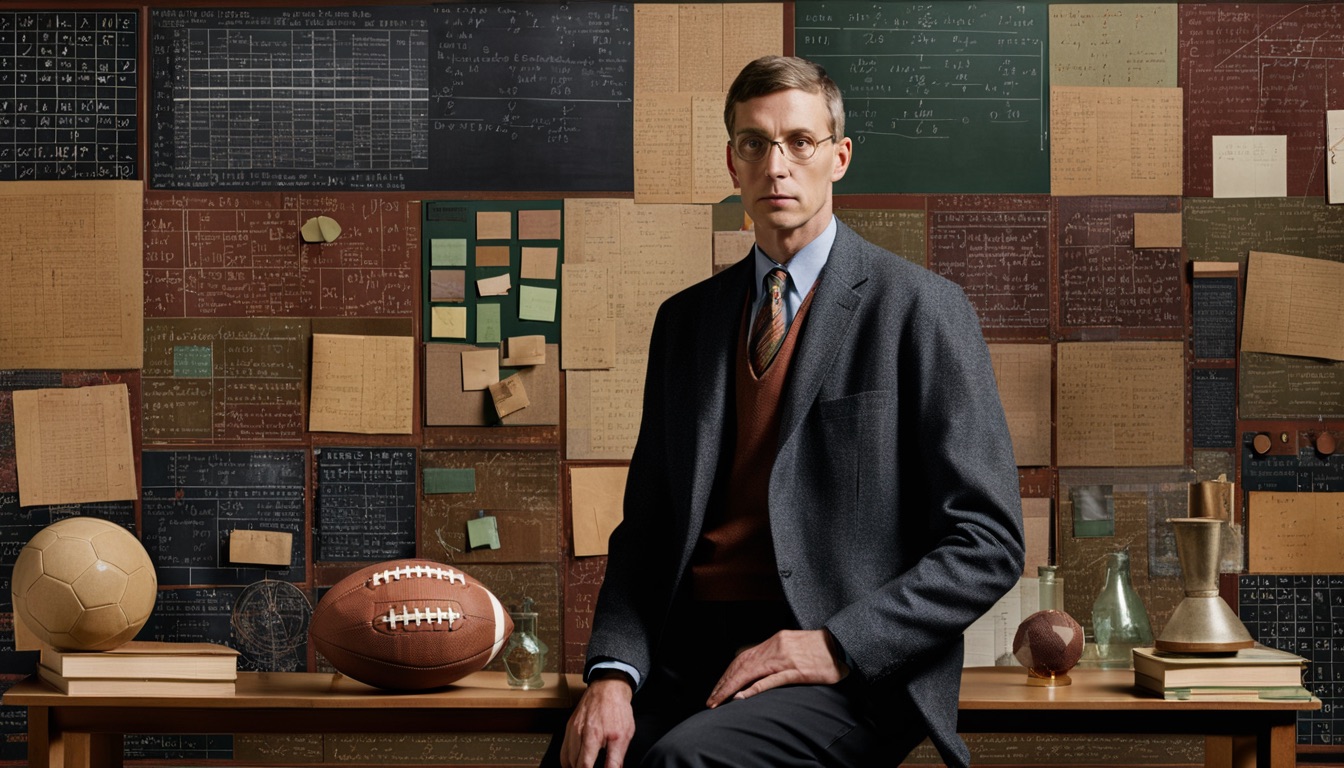
In This Issue
- What’s AI Doing for Companies Like Mine?
- Learn what General Motors, RXO, XPO, Phlo Systems, and Amazon Prime Video are doing with AI.
- Prompting
- Learn how to use ChatGPT Custom Instructions to automatically apply the How to Prompt best practices to every chat.
- What Can AI Do for Me?
- Learn how to use Custom Instructions to turn GPT-4 into a marketing advisor following the lessons of Seth Godin.
Intro
This week’s Splendid Edition will hopefully show you a glimpse of the astonishing power of large language models thanks to a rather understated new feature of ChatGPT: Custom Instructions.
The things I share with you every week, I research and test them just before writing about them. In a sense, this newsletter is like a journal of experiments that I share with you in almost real-time.
And occasionally, I discover things I’m really excited to share with you. This is one of those weeks.
Alessandro
You need a paid membership to read this content.