Small language models demonstrate unexpected effectiveness with Phi-2
December 12 2023
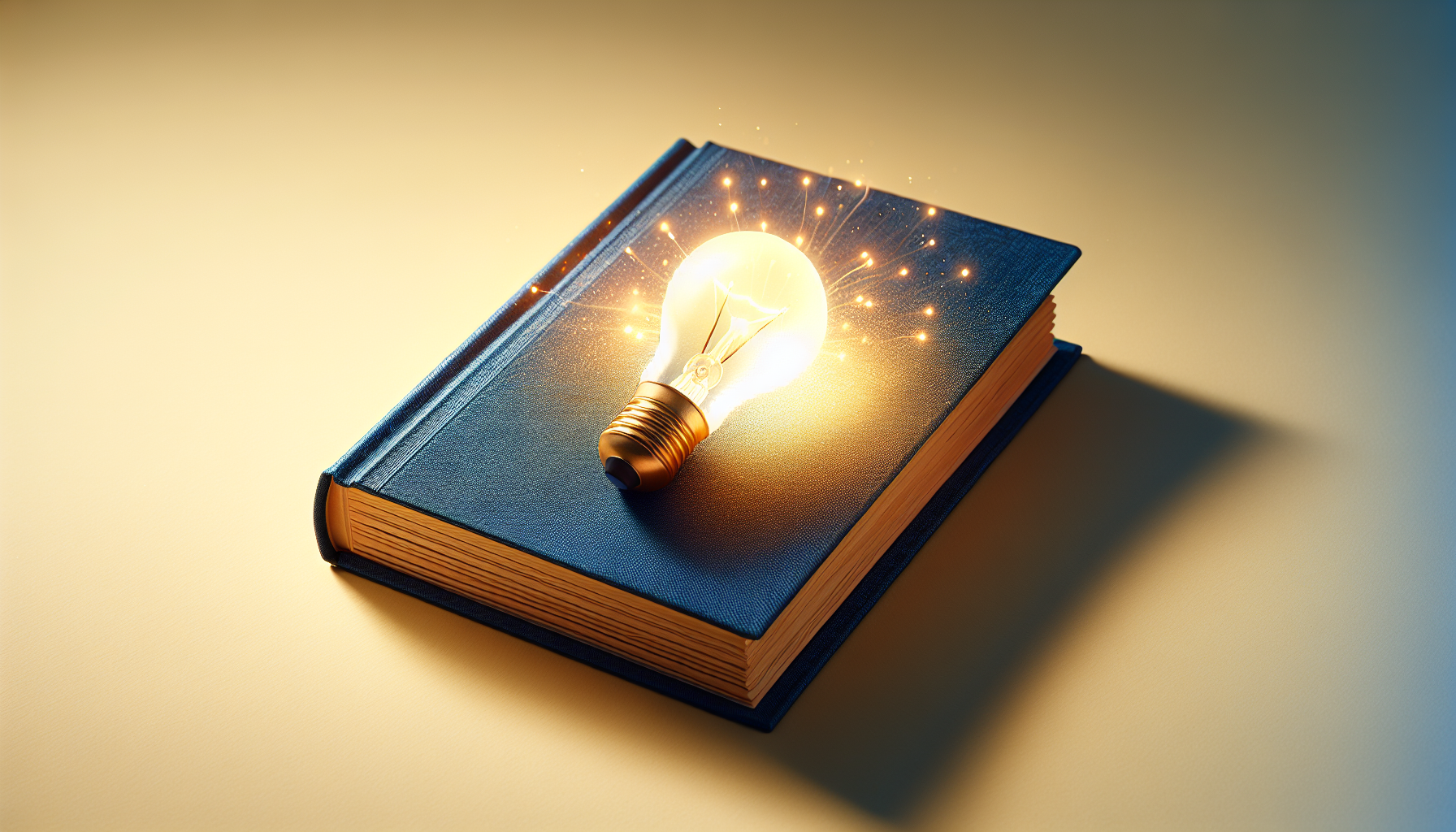
The Microsoft Research Machine Learning Foundations team has introduced a series of efficient small language models (SLMs) labeled “Phi,” with the latest being Phi-2, a 2.7 billion-parameter model that exhibits advanced reasoning and language understanding amidst base models with less than 13 billion parameters. Phi-2 rivals models up to 25 times its size on benchmarks due to innovations in model scaling and selective training data, particularly focusing on “textbook-quality” data for enhanced common sense reasoning and general knowledge. Phi-2’s compact size positions it as a versatile tool for research. Unlike some larger models, it does not use reinforcement learning from human feedback or instruct fine-tuning, but still shows better behavior in toxicity and bias due to its tailored data curation technique. Microsoft promotes exploration and development using Phi-2 by making it accessible in the Azure AI Studio model catalog.
Back to Breaking AI News
What does it mean?
- Machine Learning: A field of computer science that enables computers to learn from experience and data without being explicitly programmed for specific tasks.
- Small language models (SLMs): Machine learning models designed to understand, interpret, and generate human language but on a smaller scale than typical language models.
- Parameter: A configuration variable that is internal to the model and whose value can be estimated from the provided data. It helps determine the output of the model for a given input.
- Model scaling: The process of increasing the size of a machine learning model in terms of its parameters, data, or computational resources to improve its performance.
- Selective training data: The use of a specific subset of data chosen with particular criteria for training a machine learning model in order to improve its performance on certain tasks.
- "Textbook-quality" data: Data that is considered high-quality, reliable, and often used for educational purposes, similar to the information found in textbooks.
- Common sense reasoning: The ability of a machine learning model to make judgments and decisions that are similar to what a human using "common sense" would make.
- General knowledge: Information that is widely accepted and known by many people, which can be helpful for a language model to understand context and make inferences.
- Reinforcement learning from human feedback: A training method for machine learning models where the model is refined based on feedback received from human interactions.
- Instruct fine-tuning: A process of adjusting a pre-trained model with additional training to meet specific instructions or tasks.
- Toxicity: In the context of language models, it refers to the degree to which the content generated by a model can be offensive or harmful.
- Bias: A machine learning model's tendency to make unfair decisions or predictions based on prejudiced assumptions in its training data.
- Data curation technique: The methods used to collect, manage, and organize data to ensure it is suitable for training a machine learning model.
- Azure AI Studio: A cloud-based environment designed by Microsoft for training, deploying, and managing machine learning models.
- Model catalog: A collection or repository of pre-built machine learning models available for use or further development.
Does reading the news feel like drinking from the firehose?
Do you want more curation and in-depth content?
Then, perhaps, you'd like to subscribe to the Synthetic Work newsletter.
Many business leaders read Synthetic Work, including:
CEOs
CIOs
Chief Investment Officers
Chief People Officers
Chief Revenue Officers
CTOs
EVPs of Product
Managing Directors
VPs of Marketing
VPs of R&D
Board Members
and many other smart people.
They are turning the most transformative technology of our times into their biggest business opportunity ever.
What about you?
Do you want more curation and in-depth content?
Then, perhaps, you'd like to subscribe to the Synthetic Work newsletter.
Many business leaders read Synthetic Work, including:
CEOs
CIOs
Chief Investment Officers
Chief People Officers
Chief Revenue Officers
CTOs
EVPs of Product
Managing Directors
VPs of Marketing
VPs of R&D
Board Members
and many other smart people.
They are turning the most transformative technology of our times into their biggest business opportunity ever.
What about you?