August 26, 2023
Splendid Edition
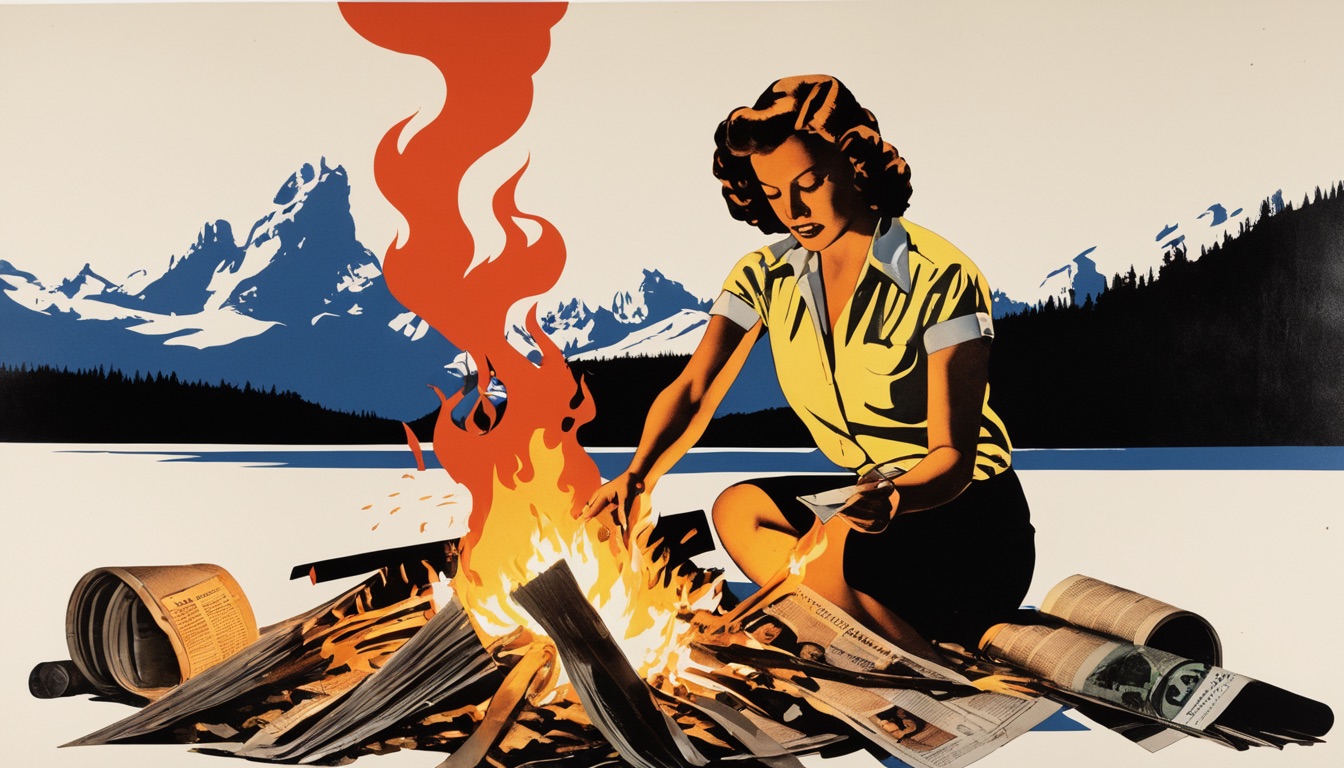
In This Issue
- What’s AI Doing for Companies Like Mine?
Learn what Estes Express Lines, the California Department of Forestry and Fire Protection, and JLL are doing with AI. - A Chart to Look Smart
McKinsey offered a TCO calculator to CIOs and CTOs who want to embrace generative AI. Something is not right. - What Can AI Do for Me?
How I used generative AI to power the new Breaking AI News section of this newsletter. You can do the same in your company. - The Tools of the Trade
A new, uber-complicated, maximum-friction, automation workflow to generate images with ComfyUI and SDXL.
Intro
As I mentioned in the intro of this week’s Free Edition, starting this week, the Splendid Edition gains exclusive access to the A Chart to Look Smart section.
This section will continue to be about reviewing high-value industry trends, financial analysis, and academic research focused on the business adoption of AI. Going forward, there will be even more emphasis on hard-to-discover academic research that might give your organization an edge.
Also, the section Screwed Industries changes name to What’s AI Doing for Companies Like Mine?
Let me know what you think about these changes.
Alessandro
You need a paid membership to read this content.