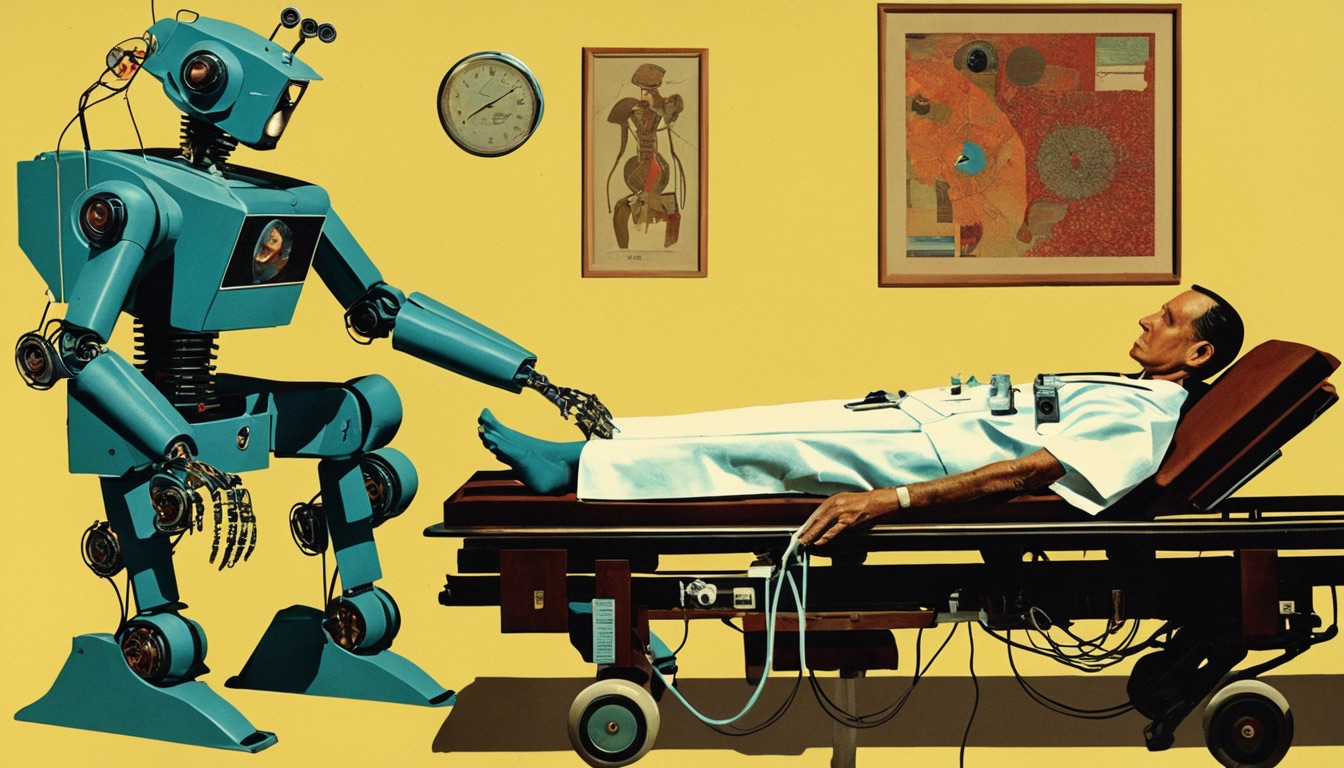
- Intro
- What is software?
- What’s AI Doing for Companies Like Mine?
- Learn what DoorDash, Kaiser Permanente, and BHP Group are doing with AI.
- A Chart to Look Smart
- Researchers have used large language models to analyze the impact of corporate culture on financial analyst reports. Can this approach be used elsewhere?
- The Tools of the Trade
- Let’s use a voice generation tool to produce an audio version of Synthetic Work
Tim Sweeney, the controversial founder and CEO of Epic Games, during a recent Q&A said:
Programming languages are the bottleneck for developing software.
Emad Mostaque, the controversial founder and CEO of Stability AI, commented on that, saying:
Won’t be needed in a few years which begs the question what is software.
What if they are right?
In multiple issues of Synthetic Work, I suggested that the future of application development is not a programming language, but a natural language. And this is one of the biggest ideas introduced by generative AI.
Are you leading a software company? If yes, are you thinking about this?
Alessandro
What we talk about here is not about what it could be, but about what is happening today.
Every organization adopting AI that is mentioned in this section is recorded in the AI Adoption Tracker.
In the Foodservice industry, DoorDash is launching an AI that automatically answers all calls from the customers.
Aisha Malik, reporting for TechCrunch:
The company’s 2023 Restaurant Online Ordering Trends Report reveals that one in five customers prefer to order takeout via phone, but up to 50% of customer calls are left unanswered, which results in potential revenue losses.
…
DoorDash is coupling AI with live agents to ensure customer calls are answered with little to no wait, enabling operators to capture the unmet customer demand. During peak times at restaurants, AI will answer calls, allowing employees to focus on in-store customers.
…
Customers will have a personalized voice ordering experience in multiple languages with no missed calls or long wait times. Returning customers will be able to quickly reorder their favorite meal. Live agents will be available to jump in to support customers at any time.
If you are interested in reading their report, you can find it here.
Once the voice side of generative AI becomes mature enough (we are close, but not there yet), we should expect a future where all calls to any customer service in any industry are answered by AI, by default. Human operators would be just a backup for edge cases. Which implies that employers would have to hire way fewer people to answer the phones.
In the Health Care industry, Kaiser Permanente is using AI to detect a deterioration in patients’ health, to sift through the messages between patients and physicians, and to identify high-risk features in breast mammograms.
From the American Medical Association (AMA) interview with Vincent Liu, MD, MS, a senior research scientist and regional medical director of Augmented Clinical Intelligence at Kaiser Permanente:
Advanced Alert Monitor, or AAM, is designed to identify high-risk patients in the hospital who are at risk for adverse events like impending admission to the ICU or unexpected death. So it’s been known for some time that these patients are at risk. And the question was, could we leverage AI algorithms and machine learning in order to really prevent and respond to these patients?
So over the past several years, we’ve used millions—hundreds of millions of data points from our hospitalized patients and granular EHR data, things like lab values, vital signs, other key clinical data to develop a machine learning algorithm that worked with good accuracy to predict patients at risk for deterioration in the next 12 hours, so early enough to actually intervene and hopefully prevent.
…
Our results were published a couple of years ago in the New England Journal of Medicine, which showed that the implementation of AAM within this entire program, working closely with clinicians, reduced mortality, reduced the rate of ICU transfers and is estimated to save as much as 500 lives per year across our hospitals.
…
As you know, AI can become a specialist in many different domains. So one example would be using natural language processing to examine the contents of messages between patients and physicians. As we all know, physicians are increasingly being overwhelmed by the volume of these messages and finding it challenging to really understand how do we sort them, the ones that are most urgent, the ones which really don’t need a physician’s attention, and then probably the ones in between where it’s not clear and it needs to initiate a more complex conversation.So over the past several years, a team here at KP has been working on using natural language processing to actually examine these notes and begin to put them into buckets that have a very, again, strongly paired workflow. So whether it’s workflow related to COVID vaccination or paxlovid or when are these facilities—what are the hours of these facilities or routine prescription refills, making sure that those get sorted appropriately, passed on to the right people and those concerns are addressed.
…
investigators here at KP have looked at thousands or tens of thousands of images like breast mammograms and found that computer vision algorithms can identify high risk features even within screening mammograms that were called normal by radiologists. And when we pair those with workflows, there’s the potential to increase the identification of patients who may be at risk for breast cancer from 20% using traditional approaches to as much as 60% to 70% using, again, this computer vision augmentation.And that unlocks a lot of opportunities, personalized screening recommendations for people at risk for breast cancer, targeted outreach for patients who are overdue for screening, or even the potential to rapidly look at images on the same day and avoid having patients come back for a second visit, escalate those images for immediate review and have the patient stay there to get their care in one visit.
So far, thanks to Synthetic Work’s AI Adoption Tracker, we have identified 4-5 main use cases for AI in the Health Care industry. But there’s a lot more coming:
Unger: So I’m curious what’s next on the agenda in terms of new capabilities or new tools that you’re hoping to implement over the next couple of years.
Dr Liu: Yeah, I mean, there’s so much exciting stuff on the frontier. I can speak to what I think is almost mature or really entering into workflow. Computer vision, whether that’s radiology, dermatology, pathology, EKGs or EEGs, we’ve seen just a large expansion of these technologies and then their integration into products. And so I think that’s a very tangible next step. And a lot of those conversations are happening in partnership with our industry and other medical technology companies.
I think there’s been huge excitement about large language models. Is there a way that we can leverage these very, very complex and large statistical models to improve communication with our patients? Can they auto draft our notes or our secure messages? I think there’s potential there. But we have to use it cautiously.
Again, thanks to the AI Adoption Tracker, we know that multiple health care organizations are already using GPT-4 to draft notes and messages. The adoption is growing, just not evenly, with some health care providers being more aggressive than others.
In the Mining and Resources industry, the BHP Group is using AI to increase the recovery of copper from one of the biggest mining sites in the world.
From the official announcement:
A new collaboration between BHP and Microsoft has used artificial intelligence and machine learning with the aim of improving copper recovery at the world’s largest copper mine.
The use of new digital technology to optimise concentrator performance at BHP’s Escondida operation in Chile is expected to improve copper recovery.
…
BHP estimates the world would need to double the amount of copper produced over the next 30 years, relative to the past 30, to keep pace with the development of decarbonisation technology such as electric vehicles, offshore wind and solar farms assumed under its 1.5 degree scenario.
…
By using real-time plant data from the concentrators in combination with AI-based recommendations from Microsoft’s Azure platform, the concentrator operators at Escondida will have the ability to adjust operational variables that affect ore processing and grade recovery.BHP is a top three global producer of copper and has the largest copper endowment of any company globally.
On top of this, BHP is also using self-driving trucks, as disclosed in their 2023 report:
We’re using autonomous trucks at some of our sites across Western Australia and Queensland and extending this to Spence and Escondida. At Jimblebar and Newman, truck automation has resulted in a 90 per cent reduction in heavy vehicle safety risks.
You won’t believe that people would fall for it, but they do. Boy, they do.
So this is a section dedicated to making me popular.
A new research titled Dissecting Corporate Culture Using Generative AI – Insights from Analyst Reports is particularly interesting for all of you leading a large enterprise, especially if it’s on the stock market.
This research is interesting for two reasons:
- The researchers found out that discussing the corporate culture with financial analysts influences their stock
recommendations and target prices. - To reach this conclusion, they used large language models to analyze 2.4M analyst reports in the last 20 years, showing yet another way AI can transform finance, sharing a lot of their strategies.
On the first point:
We show that changes in business strategy, doing M&As, management changes, adopting disruptive technology, experiencing regulatory issues, or targeted by shareholder activism are all positively and significantly associated with cultural changes. In terms of notable consequences, we show that firms with a strong culture are negatively and significantly associated with emissions and workplace safety violation cases, confirming the culture-consequence link uncovered in the casual relation extraction analysis.
…
We conclude that analyst reports offer insights into the mechanisms through which culture affects business outcomes and that analysts’ research on culture contributes to the observed culture-firm value link.
On the second point:
Our study highlights both the potential and limitations of generative AI in the context of financial text analysis. We show that generative AI is highly effective in complicated information extraction tasks. However, there are unique considerations and design elements that need to be addressed in order to harness the full potential of these models. For instance, a step-by-step. chain-of-thought prompting strategy is beneficial for extracting causal relations – a task that requires high-level reasoning.
Furthermore, all generative AI models have intrinsic context length limitations, making it impossible to ask them to analyze a report in its entirety.
We demonstrate that feeding smaller segments related to corporate culture, while allowing for dynamic augmentation of input segments by searching for relevant information from a full report, can enhance the overall capability of these models.
Finally, our approach of extracting causal relations in analyst reports goes beyond the boundaries of surface-level text classification tasks common in finance, accounting, and economics applications. Our method enables a deeper exploration of embedded narratives within financial documents, which allows for the distillation of knowledge in a human-interpretable, structured form that captures discrete and sometimes abstract concepts (e.g., corporate culture, people, or events) and their relationships. By uncovering causal relations between entities that might otherwise remain obscure, the insights gained open new avenues
for research in areas such as risk management, predictive analysis, and automated reasoning.
Now. Are you thinking what I am thinking?
What if the mentions of corporate culture equally affect technology analyst reports? Wouldn’t you want to know?
If I were leading the analyst relations team of a large enterprise, I would immediately start a project to analyze with GPT-4 the corpus of both financial and technical reports produced by the analyst community about my products.
You’d want to ingest not just the earnings call transcripts, but also the notes or the recordings (whenever possible) of the interactions between analysts and your executives and product managers to understand better the correlations.
I was an industry analyst in a past life, and I can guarantee you that who speaks to the analysts and the things they say, no matter how unrelated to the product, influence the analysts’ perception of the company and its products.
Not only that. I can guarantee you that the analysts’ perception also changes in terms of optimism toward the company’s future, and the capability to compete.
An analyst who believes in a particular spokesperson, or who admires that person, will more likely see a bright future for the company, even if there’s no evidence to support that feeling.
What might happen now is that companies start to measure and A/B test what they say during these interactions, optimizing their statements to maximize analyst sentiment, price targets, and product ratings.
Among the other things, many of the techniques we listed in How to Prompt can be used to achieve the task.
If you don’t do that, I’m confident some of your competitors will.
The time of synthetic voice has come.
If you read this week’s Free Edition of Synthetic Work, and you bother to listen to a rap song I linked to, you are starting to realize that the quality of synthetic voices has reached a quality suitable for almost any application.
To further demonstrate this point, this week, we have an audio version of the Free Edition of Synthetic Work.
Before I tell you what’s the best tool to use, and how I used it, let me remind you of the challenge we are grappling with here.
As long-time readers might remember, my benchmark for synthetic voices in terms of quality and expressiveness is defined by the two voices I used in the promo of my forever-in-progress podcast called Fake Show.
If you don’t remember what we are talking about, you might want to hear those voices again, paying attention to the emotions that I was able to convey with them:
These voices were generated with an open source generative AI model called Tortoise, trained two years ago by a brilliant engineer named James Betker on his home computer. It was not exactly a consumer-grade computer, of course, as it featured 4 GPUs, but it was not a supercomputer either.
I could do very special things with a machine like that, but I’m digressing.
The name of the model James developed is Tortoise, and it set the quality bar for every single voice generation project or service out there. People in the AI community don’t like to mention it, but they all know that they have to beat that quality.
James was immediately hired by OpenAI and abandoned Tortoise. But being open source code, anybody can access it, including yours truly.
So why isn’t every service on the planet using Tortoise to generate voices?
Firstly, because it’s painfully slow to be commercially viable. Secondly, because you can only generate a very short sentence at a time. You can’t simply pass a long text to the model and get a long audio file back. You have to generate each sentence separately, and then stitch them together.
It’s OK for a homemade podcast like Fake Show, or to generate a few voices that will rarely have to say new things (like the announcements in the London Underground, for example), but it’s not OK if you need to generate new text every minute for millions of users, or if you have weekly deadlines.
So, these services and end-user organizations, perhaps like yours, are using a wide range of other open models. They work OK, but they lack the expressiveness of Tortoise, sounding quite monotone.
Once you hear what you can do with Tortoise in the Fake Show promo, it’s hard to settle for other voices.
All of this is changing, tho, as the AI community has now managed to unlock new, exceptional generative AI models.
In the last few weeks, the top two commercial services for voice have released their v2 models and they are remarkable. These two are PlayHT and ElevenLabs.
These models offer a level of expressiveness that is finally very close to what you’d get with Tortoise, generating voices at a greater speed. And, crucially, some of them also offer a way to control emotions, via a prompt, just like the one one you use for large language models or text2image models.
Finally, and critically for many companies, these new models are available in a wide range of languages, opening the door to commercial services across the globe.
So, it’s time to adopt some emerging tech!
While, in my opinion, there is a difference in terms of quality between the new PlayHT 2.0 model and the new Eleven Multilingual v2 model, you have to take into account that quality is not the only factor to consider when choosing a model.
A voice model might be better in quality but be underwhelming when it comes to respecting the pauses between sentences. In that case, you are forced to do a lot of extra editing of the text, and the model ends up costing you a lot more than you originally expected.
Similarly, a voice model might sound great with ordinary words but struggle with acronyms and words in other languages that might be peppered in your text, like French or Italian. In that case, you’d have to go for untold acrobatics to make the pronunciation work correctly, spending a lot of extra time on editing and money on speech generation.
I had these exact problems with the PlayHT model in the past. Their new v2 model sounds exceptional, but it’s still in beta, and since my experience with them was a bit frustrating, I’ll pass it until it reaches the general availability phase.
The quality of the new ElevenLabs model is remarkable, too. And I’ve found no trace of the issues I had with PlayHT.
Hence, to demonstrate how far we’ve come with voice generation, this week I used the ElevenLabs Eleven Multilingual v2 model to generate the audio version of the Free Edition of Synthetic Work.
By the way, in Issue #9 – The Tools of the Trade, we discovered how 88Nine Radio Milwaukee is using Eleven Labs technology to power a synthetic radio host.
So. How did I do this?
Once signed up for the service (you want at least the Creator plan, to be sure you have a high-quality audio output), you could head to the Speech Synthesis section of the website where you can pick or customize a premade voice.
If you do that, be sure to select their Eleven Multilingual v2 model:
Then, you can pick a voice from the list of available ones:
At this point, you can keep the voice as is, or tweak several parameters:
Changing these parameters is the key to generating a synthetic voice that is unique for your product or service without cloning a human voice with a voice actor. However, if you increase some of these values to make the voice more expressive, you might end up with artifacts in the audio: background noises, spoken words that don’t reflect your text, etc.
So, be sure to experiment a lot with the synthetic voice you end up generating.
If you are not too concerned about the originality of the voice, you could go with the default voices or, as I recommend, explore the voices that other ElevenLabs customers have created and shared with the community.
This wonderful feature is in the Voice Library section of the website and, if nothing else, it’s very helpful to understand what you can achieve with the service:
The voices you find here are generated using the same steps we saw above. The only difference is that, once you have tweaked one of the default voices, you give it a description and a series of attributes, and then you share your custom voice.
Whether you have modified a default voice from the Speech Synthesis section or you have selected a community voice from the Voice Library, you end up with a custom voice saved in your Voice Lab section.
Depending on your plan, you get to save a certain number of voices.
ElevenLabs also offers two voice cloning services: Instant Cloning and Professional Cloning. The latter promises to generate a synthetic voice indistinguishable from the original one.
A professionally cloned voice takes 2-4 weeks on average to be produced, as the company performs a full training session for their foundational AI model with the audio sample that you have provided.
If Synthetic Work readers are interested in an audio version of the newsletter, I might attempt to clone my voice with the Professional Cloning service, giving ElevenLabs the right to use my voice to say despicable things in perpetuity. At least, you’d have a sense of the maximum quality that is achievable with their model.
Whichever voice cloning service you end up using, it will end up in the Voice Lab section, too.
At this point, all you have to do is go back to the Speech Synthesis section, select the voice you want to use, and paste the text you want to generate.
Depending on your subscription level, you can generate chunks of 2,500 characters or 5,000 characters. No more. So, there’s still a lot of manual work involved.
The alternative is to subscribe to their Independent Publisher plan, which allows you to generate text via an API, automating the whole process.
During this test, I discovered that you can upload text that contains HTML tags and ElevenLabs will safely ignore most of them. Of course, it will consume some of your character quota, but it might speed up your workflow. But, in some cases like the summary of the Issue, the use of HTML tags improved the situation, forcing the synthetic voice to pause at the right places.
Overall, it was a very laborious process that took many hours.
In part, because I had to re-generate the same segment multiple times as I learned how the synthetic voice would react to things like quotes, ellipses, HTML tags, and more. Once learned that, it was a straightforward job.
In part, because I had to edit the voice segments together in a somewhat final product, which is here (and at the top of the Free Edition):
Let me know what you think by replying to this email.
As you’ll find out, especially if you compare what you hear with what you read, the result is not perfect. The synthetic voice still struggles a bit in giving the right intonation to questions, and certain pauses are either too long or too short.
Additionally, it’s impossible to understand when a quote ends and my writing starts.
You could say that the whole speech could be a little more expressive. While true, it’s worth remembering that many human beings are significantly less expressive than the voice I selected.
Overall, I think I obtained an impressive quality. In one year, synthetic voices might become truly indistinguishable from human voices.
The most important thing that you need to keep in mind is this: the whole process can be automated.
We are entering uncharted territory for our species, where various incarnations of generative AI, producing text, images, voices, and soon music and entire films, will be able to churn out entertainment and information at a speed that is impossible to match with human labor.