October 14, 2023
Splendid Edition
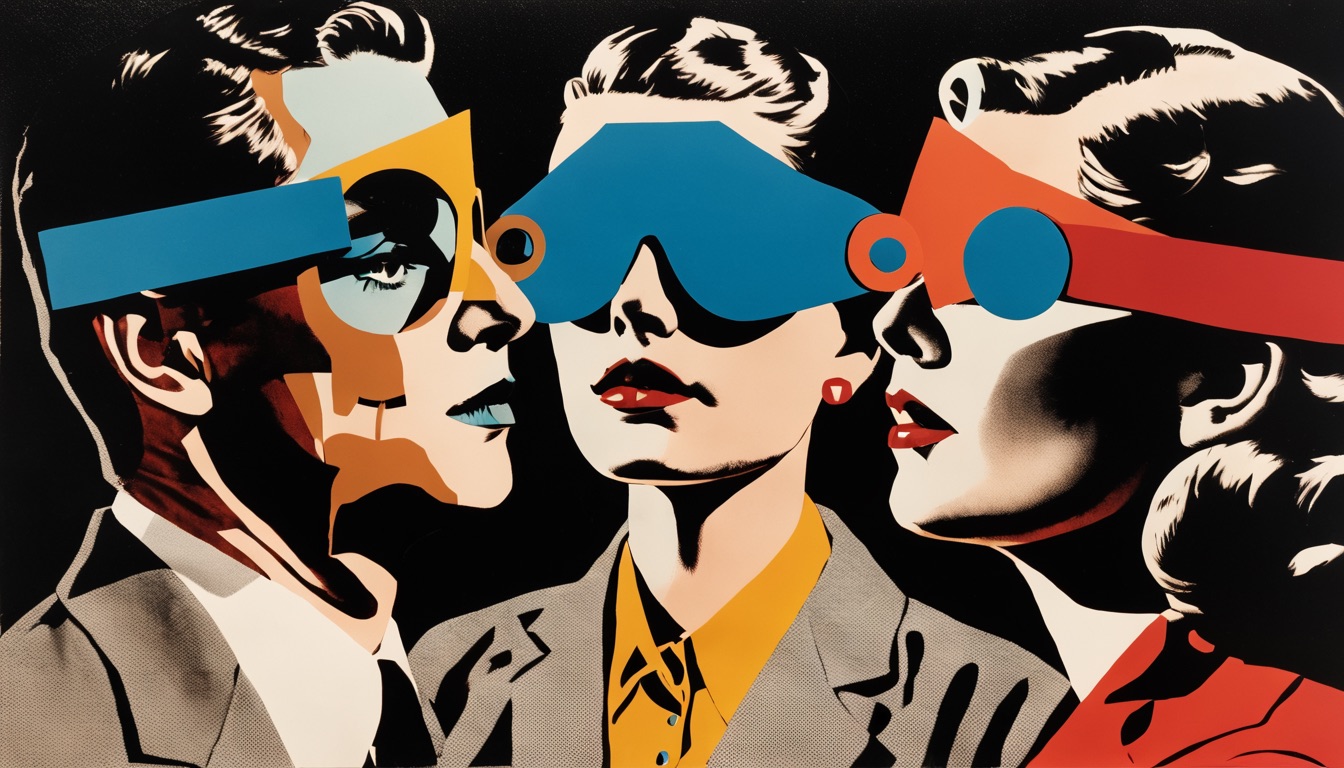
In This Issue
- What’s AI Doing for Companies Like Mine?
- Learn what BBC, The Washington Post, and Thomson Reuters are doing with AI.
- A Chart to Look Smart
- What can we do by training an AI with the lifetime of the most intelligent person on Earth, educated to be a business/science/engineering/etc. leader, and recorded as he/she goes through life and is successful?
- What Can AI Do for Me?
- Here’s how the new GPT-4V might transform customer support.
- The Tools of the Trade
- Finally, a tool I can recommend to organize ChatGPT chats.
You need a paid membership to read this content.