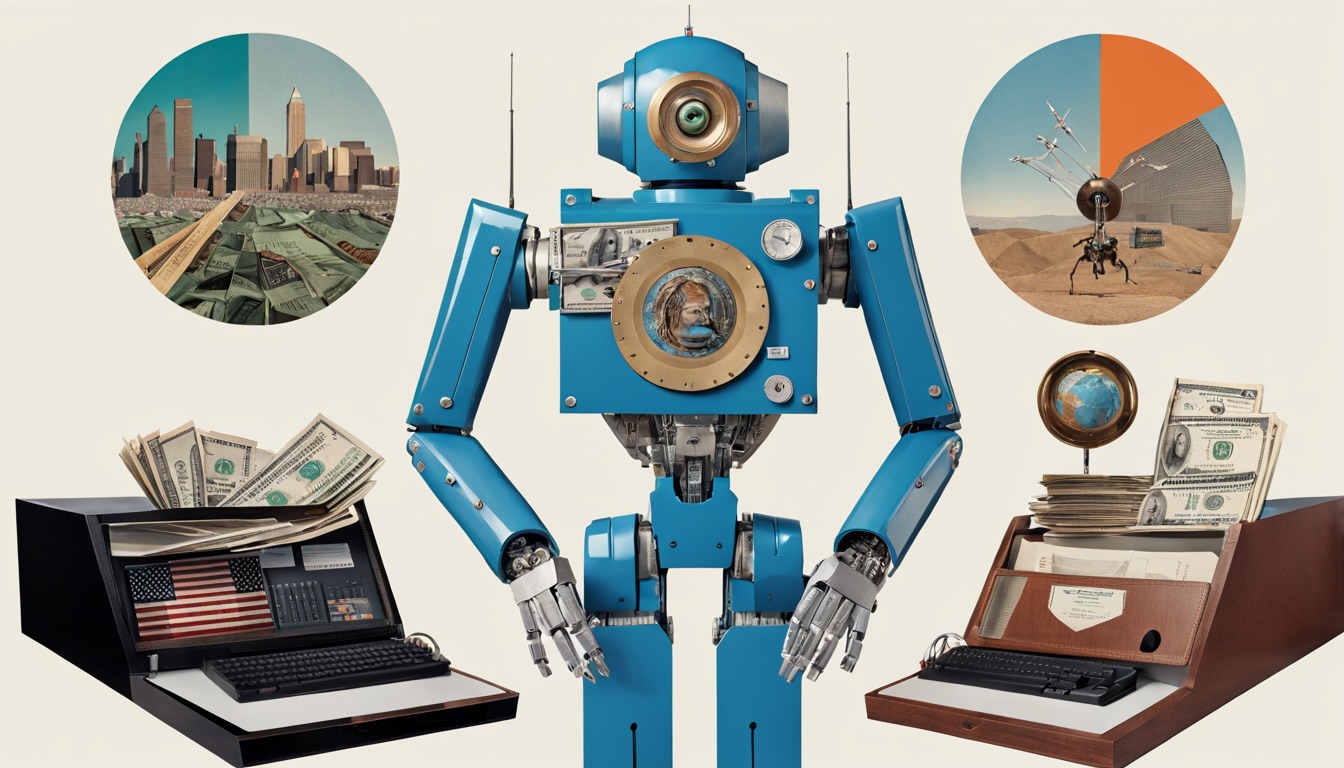
- Intro
- Happy New Year.
- What’s AI Doing for Companies Like Mine?
- Learn what Allen & Overy, Addleshaw Goddard, Travers Smith, Macfarlanesand, H&R Block, and Hiscox are doing with AI.
- A Chart to Look Smart
- How AI for financial management is being pushed by CFOs in midsized companies.
- Prompting
- 26 tested recommendations to improve your prompting with GPT-3.5-Turbo, GPT-4, and LLaMA 2
- The Tools of the Trade
- OpenBB Terminal, the open source alternative to Bloomberg Terminal, is now powered by AI
It’s been a privilege to serve you all for the last 42 weeks.
We close the year with 130 companies and 150 unique use cases in the AI Adoption Tracker.
Without your support, Synthetic Work would not exist.
Thank you, and Happy New Year.
Alessandro
What we talk about here is not about what it could be, but about what is happening today.
Every organization adopting AI that is mentioned in this section is recorded in the AI Adoption Tracker.
In the Legal industry, Allen & Overy is using AI to power a contract negotiation tool, Addleshaw Goddard is testing multiple AI solutions for tasks like document drafting and summarising case law.
Cristina Criddle and Suzi Ring, reporting for the Financial Times:
The UK-headquartered group, in partnership with Microsoft and legal AI start-up Harvey, has developed the service which draws on existing templates for contracts, such as non-disclosure agreements and merger and acquisition terms, to draft new agreements that lawyers can then amend or accept.
The tool, known as ContractMatrix, is being rolled out to clients in an attempt to drive new revenues, attract more business and save time for in-house lawyers. A&O estimated it would save up to seven hours in contract negotiations.
More than 1,000 A&O lawyers are already using the tool, with five unnamed clients from banking, pharma, technology, media and private equity signed up to use the platform from January.
In a trial run, Dutch chipmaking equipment manufacturer ASML and health technology company Philips said they used the service to negotiate what they called the “world’s first 100 per cent AI generated contract between two companies”.
…
David Wakeling, A&O partner and head of the firm’s markets innovation group, which developed ContractMatrix, said the firm’s goal was to “disrupt the legal market before someone disrupts us”.“If we look at the impact of AI in law, it is happening and it’s likely to happen in a pretty big way in the next few years, and we are seeing it as an opportunity,” he added.
The firm is also planning to offer the service to the clients it gains from its $3.5bn merger with US outfit Shearman & Sterling, said Wakeling, which is due to complete by May.
…
A&O would not detail specific financial terms around the contract negotiation tool but said clients would pay an annual subscription fee per licence, with a minimum purchase of five users. The law firm is aiming to have subscriptions with hundreds of companies by the end of next year.
…
Wakeling said that hallucinations could happen with ContractMatrix, but they were significantly reduced because of the templates its underlying technology had been trained on. He added that client data was also not used to train the AI models that underpin the software, and inputs and outputs are encrypted.
Allen & Overy was featured in the second issue of this newsletter, at the beginning of 2023. It seems fit to end the year mentioning them again.
As you read/will read in this week’s Free Edition of Synthetic Work, the legal industry is struggling to contain the growing (and occasionally reckless) adoption of AI.
Allen & Overy has been an inspiring example and has pushed many other law firms to experiment with large language models, including Addleshaw Goddard, Travers Smith, and Macfarlanes, which have rolled out generative AI pilots internally.
From the Addleshaw Goddard’s corporate blog:
International law firm Addleshaw Goddard has chosen CoCounsel, Spellbook, and Microsoft Copilot alongside its own internally developed AGPT platform, as its suite of Generative AI solutions. This follows a year of research by the firm’s Innovation & Legal Technology leadership, led from the team’s R&D pillar managed by Mike Kennedy. Over 50 technologies were reviewed, with five taken forward to trials and pilots involving 150 volunteers across the firm.
…
The pilots identified more than 100 potential use cases across all of our practice areas, with common functions including producing first drafts of content for review e.g. contract clauses, document review, summarising case law, contracts and articles, overcoming writer’s block, translation, research and information-gathering.
…
AGPT is AG’s own internally developed AI-powered platform that enables users to run standard queries, upload documents to be queried and reviewed, and translate documents. Built within our secure Microsoft Azure environment to ensure nothing leaves the firm’s networks, it was created in close collaboration between our Innovation & Legal Technology and IT teams over the past half year.
From the Travers Smith’s corporate blog:
Created by the firm’s pioneering Legal Technology team, YCNBot (which stands for Your Company Name e.g. TSBot), is available for anyone to download and deploy free of charge through an open source license, enabling, law firms and other organisations to replace the consumer facing ChatGPT with a chat-bot that plugs into Enterprise API’s of Microsoft and OpenAI. This ensures essential enhanced controls around compliance, security and data privacy for businesses which handles confidential, sensitive and Personal Identifiable Information (PII).
TSBot has been rolled out across at Travers Smith, with lawyers and business services employees encouraged to learn, experiment, and provide feedback to the Legal Technology team. It will not be used for any direct client work.
Travers Smith developed the platform with guidance and support from 273 Ventures, led by Mike Bommarito and Dan Katz. The two companies will continue to develop and enhance YCNBot, building in further safeguards proposed by regulators as the technology continues to evolve. The latest version includes enhanced privacy, the ability to submit feedback, and an analytics view for administrative users.
From the Macfarlanes’ corporate blog:
Macfarlanes is to roll out HarveyAI (Harvey), the generative AI tool designed specifically for legal work, as part of its AI strategy. The decision to be an early adopter positions the firm at the forefront of the developing use of generative AI in the legal sector.
…
The Harvey roll out follows a pilot programme undertaken earlier in the summer, carried out by more than 70 fee earners and knowledge lawyers across the firm.
…
It can answer general legal questions, as well as undertake drafting, providing new and efficient solutions which would not be possible without technological innovation. All outputs will be carefully monitored and reviewed by Macfarlanes lawyers.
In the Financial Services industry, H&R Block is using AI to help its customers with tax preparation.
Emilia David, reporting for The Verge:
AI Tax Assist, accessed through paid versions of H&R Block’s DIY tax software, lets users ask questions on tax rules, possible exemptions, and other tax-related issues. It also points people to a human tax expert for more personalized advice, though the company said it is working on supporting AI-powered personalized advice as well.
In a demo, the company showed how users with complicated tax filing statuses — say a gig worker — can start their tax returns. They can then ask AI Tax Assist for information like, “What can I deduct as business expenses as an Uber driver?” The AI will provide a list of allowed deductions, and the user can choose to use that list or not in the final filing.
H&R Block will show a banner informing users that the feature is powered by AI and offer sample prompts.
…
Chris Linderwell, vice president of consumer tax products at H&R Block, said during a briefing that many users often turn to Google when they’re confused about how to file their taxes. AI Tax Assist is meant to encourage users to stay on H&R Block’s platform when seeking tax answers instead.
…
H&R Block built AI Tax Assist with models from OpenAI and Microsoft. The company emphasized that it trained only on H&R Block’s library of tax laws and refined with the help of its many accountants, lawyers, and other tax professionals. It said the model did not pull from the internet. Linderwell said the feature was built to only answer with specific H&R Block content. H&R Block, which does still operate brick-and-mortar tax services, hopes that AI Tax Assist feels a lot like speaking to one of its human accountants. If people have concerns over the AI-provided answers, the company said their team of live human experts can also provide their services via phone.
…
Of course, there is always the possibility of hallucinations that produce false information — and in tax preparation, the stakes are unusually high. While no company can yet guarantee AI will not hallucinate, H&R Block said users can double check information with a human. It also has a team that monitors the model for accuracy and that it behaves the way it was designed.
There are two problems with this approach.
The first is that, in this use case, hallucinations can have costly consequences. It’s true that looking for tax guidance on Google won’t produce more accurate results than interrogating an AI model fine-tuned on the tax code. But in the latter case, it’s easy for a user to blindly trust the chatbot rather than double-checking with a human expert.
The second problem is that the hallucination weakness can be exploited by malicious actors to evade taxes. If there’s not a verifiable track record of the interaction between the user and the chatbot, all that user has to do is pay the $35 fee to access the H&R Block paid plan, and pretend that the AI Tax Assist has provided the wrong guidance.
If caught, it will be debated in court whether the user was aware of the AI’s hallucination, whether the user was acting in good faith, where the H&R Block responsibility lies, and so on.
LLMs are not ready for unsupervised use where certainty is required.
In the Insurance industry, Hiscox is preparing to use AI to automate the underwriting process for specialist risks.
Ian Smith and Cristina Criddle, reporting for the Financial Times:
Hiscox has created an artificial intelligence model in collaboration with Google that can automate the core underwriting process for specialist risks, hailing it as a first for London’s insurance market.
The UK insurer, which plans to start using the tool next year, conducted a trial to automate the underwriting of a property sabotage and terrorism policy where it would be acting as a lead underwriter, it said on Tuesday. It would be the first time such activity had been automated, Hiscox added.
The AI tools would reduce the time spent on drawing up a quote to a few minutes rather than days and would free staff to underwrite more complicated risks, Hiscox said.
“It will allow the underwriters also to do more from a sales perspective, to travel more,” said Kate Markham, chief executive of Hiscox’s London market division. Asked about any impact on underwriter numbers, she said the business was “still hiring and planning to grow”.
…
Hiscox was likely to go live with the model in the second half of 2024, she said.“The big question for us is: do we go [with] one line of business or do we try and drop this capability across multiple lines of business — which I think is our preference,” she added.
…
AI has already been used in the sector, for example in analysing claims, but the negotiation of specialist commercial insurance contracts — where underwriters provide policies covering against everything from cyber attacks to oil spills — has remained dependent on person-to-person trading.
…
The platform, developed by a joint Hiscox-Google team, uses a mixture of Google’s generative artificial intelligence and Hiscox’s digital technologies to extract data from email submissions provided by insurance brokers and automate everything from analysis to quote.The AI digests the draft contract provided by the broker and any accompanying words and data. The decision on whether the risk falls within Hiscox’s appetite is determined by its own system. The AI tool also plays a role at the end, drafting a reply email to the broker, summarising what Hiscox has agreed to insure. The human underwriter reviews the summary before sending.
…
“We don’t want to remove the human in the chain at the moment,” said Markham. “We want to keep the underwriter so they can confirm that the models are behaving.”
As usual, statements like “we are still hiring” and “we don’t want to remove the human in the chain” are contingent on the capabilities of the AI model being used.
These policies will be certainly revisited as GPT-5 comes out.
You won’t believe that people would fall for it, but they do. Boy, they do.
So this is a section dedicated to making me popular.
Citizens Financial Group published an interesting report on how AI is being adopted by mid-sized companies and private equity firms for financial management use cases.
The survey underpinning the report was conducted in September 2023 and involved 127 CFOs in mid-size companies with annual revenues between $50 million and $1 billion and 77 financial leaders at PE firms with a fund size of less than $1.5 billion, active with mid-size companies.
It’s not a huge sample, but it’s a novel insight into the adoption of AI in the financial sector.
Let’s start with the use cases:
The second most interesting chart is about who’s driving the adoption of AI:
Given that the survey is focused on financial management use cases, it’s not surprising that the CFO is the main driver of AI adoption. Yet, the dynamic supports a concept we repeated over and over in this newsletter: AI adoption is driven by end users, as only they know the intricacies of their workflows and can identify the best opportunities for AI to improve them.
The third most interesting chart is about the concerns around the top perceived risk of AI.
When asked about that, the respondents agreed that lack of legal compliance was the top risk, but their concern about the issue varies significantly across industries:
From first-hand experience, I can say that it’s not a surprise to see the Tech industry being the least concerned about legal compliance.
The main takeaway from this report is, in my opinion, that the CFO can be a champion for AI adoption and he/she should be involved in the process of defining the target use cases for internal transformation from the beginning.
If the CFO can be convinced of the merits of AI, it will be a lot easier to fund multiple initiatives and to get the buy-in from the rest of the C-suite.
Before you start reading this section, it's mandatory that you roll your eyes at the word "engineering" in "prompt engineering".
A new research paper published this week attempts to offer formal guidance for prompting OpenAI GPT-3.5-Turbo and GPT-4, as well as Meta LLaMA 1 and 2.
It’s a precious set of 26 recommendations that is worth practicing in your daily interactions in ChatGPT or LM Studio (if you use open access models). You’ll recognize many of them from the How to Prompt library of prompting techniques we built over the last few months.
The most interesting aspect of this research is that each recommendation has been tested to measure its effectiveness for smaller LLMs (with 7B parameters), medium-sized LLMs (with 13B parameters), and large LLMs (with 70B or more parameters, like GPT-3.5 and GPT-4).
You can see how each recommendation performs differently depending on the size of the model:
(screenshot: Issue #42/Splendid/Prompting vs Model Size.png)
On average, there is a stable 50% improvement across different LLMs in terms of the quality of the output. So, it’s definitely worth paying attention to these recommendations:
- No need to be polite with LLM so there is no need to add phrases like “please”, “if you don’t mind”, “thank you”,
“I would like to”, etc., and get straight to the point. - Integrate the intended audience in the prompt, e.g., the audience is an expert in the field.
- Break down complex tasks into a sequence of simpler prompts in an interactive conversation.
- Employ affirmative directives such as ‘do,’ while steering clear of negative language like ‘don’t’.
- When you need clarity or a deeper understanding of a topic, idea, or any piece of information, utilize the following prompts:
- Explain [insert specific topic] in simple terms.
- Explain to me like I’m 11 years old.
- Explain to me as if I’m a beginner in [field].
- Write the [essay/text/paragraph] using simple English like you’re explaining something to a 5-year-old.
- Add “I’m going to tip $xxx for a better solution!”
- Implement example-driven prompting (Use few-shot prompting).
- When formatting your prompt, start with ‘###Instruction###’, followed by either ‘###Example###’ or ‘###Question###’ if relevant. Subsequently, present your content. Use one or more line breaks to separate instructions, examples, questions, context, and input data
- Incorporate the following phrases: “Your task is” and “You MUST”.
- Incorporate the following phrases: “You will be penalized”.
- Use the phrase ”Answer a question given in a natural, human-like manner” in your prompts.
- Use leading words like writing “think step by step”.
- Add to your prompt the following phrase “Ensure that your answer is unbiased and does not rely on stereotypes”.
- Allow the model to elicit precise details and requirements from you by asking you questions until he has enough information to provide the needed output (for example, “From now on, I would like you to ask me questions to…”).
- To inquire about a specific topic or idea or any information and you want to test your understanding, you can use the following phrase: “Teach me the [Any theorem/topic/rule name] and include a test at the end, but don’t give me the answers and then tell me if I got the answer right when I respond”.
- Assign a role to the large language models.
- Use Delimiters.
- Repeat a specific word or phrase multiple times within a prompt.
- Combine Chain-of-thought (CoT) with few-shot prompts.
- Use output primers, which involve concluding your prompt with the beginning of the desired output. Utilize output primers by ending your prompt with the start of the anticipated response.
- To write an essay /text /paragraph /article or any type of text that should be detailed: “Write a detailed [essay/text/paragraph] for me on [topic] in detail by adding all the information necessary”.
- To correct/change specific text without changing its style: “Try to revise every paragraph sent by users. You should only improve the user’s grammar and vocabulary and make sure it sounds natural. You should not change the writing style, such as making a formal paragraph casual”
- When you have a complex coding prompt that may be in different files: “From now and on whenever you generate code that spans more than one file, generate a [programming language ] script that can be run to automatically create the specified files or make changes to existing files to insert the generated code. [your question]”
- When you want to initiate or continue a text using specific words, phrases, or sentences, utilize the following prompt: “I’m providing you with the beginning [song lyrics/story/paragraph/essay…]: [Insert lyrics/words/sentence]’. Finish it based on the words provided. Keep the flow consistent.”
- Clearly state the requirements that the model must follow in order to produce content, in the form of the keywords, regulations, hint, or instructions
- To write any text, such as an essay or paragraph, that is intended to be similar to a provided sample, include the following instructions: “Please use the same language based on the provided paragraph[/title/text /essay/answer].”
As you can see, there are a few recommendations that we never explored. For example: #9, which I use regularly in my prompts for GPT-4.
In my research, I also noticed #4: it’s more effective to be specific about what the AI model must do rather than what it must avoid. Unfortunately, this approach is harder to follow because, for humans, it’s much easier to think in terms of absolute freedom plus some exclusions rather than a prescriptive set of instructions.
Another area we didn’t discuss much so far, but that has proven effective (to the point that even OpenAI uses it internally), is the formatting of the prompt with symbols, delimiters and line breaks. So be sure to pay attention to recommendations #8 and #17.
While these seem like a lot of rules that are hard to remember (somebody called them arcane incantations), in reality, they are not too different from the set of implicit rules that we follow in our daily interactions with our kids, partners, coworkers, etc.
For each of these interactions, we have learned, with experience, what conversation patterns work best to achieve our goals. We can do the same with AI models, as long as we are willing to experiment and be patient.
Human patience is influenced by incentives. If you don’t perceive a significant benefit from mastering the interaction with an AI model, there’s nothing I’ll ever be able to say to commit you to the practice.
One of the most promising open source projects of all time is called OpenBB Terminal. It’s an open source-based attempt to compete against the world-famous and ultra-expensive Bloomberg Terminal.
I’ve been following this project since its launch and now that the team has finally started introducing artificial intelligence, it’s time to talk about it in this newsletter.
The goal is ambitious: OpenBB intends to create a synthetic financial analyst, capable of autonomously generating a full financial report, by exposing the AI to the broad range of data reachable via the Terminal (including local and remote data sources).
The integration with OpenAI platform enabled OpenBB to reach the first three stages of the roadmap quickly.
Now they are focused on the fourth stage, a challenging one that requires their LLM to reason on how to access and combine available data to answer the user.
For example, the OpenBB Terminal user might ask something very complicated like:
Check what are TSLA peers. From those, check which one has the highest market cap. Then, on the ticker that has the highest market cap get the most recent price target estimate from an analyst, and tell me who it was and on what date the estimate was made.
and receive an answer like:
This capability will become available as part of the OpenBB Copilot which is not yet available in the alpha version of OpenBB Terminal Pro that I’m using today.
While we wait, let’s see how OpenBB infused its terminal with AI in many other ways.
First, when you look at financial news of any kind, you can ask their LLM to summarize the text or you can ask specific questions that might be answered by the text:
Financial news in brokering apps is always provided in a telegraphic way, so this might not seem like a useful feature, but oftentimes, the news also include PR announcements from this or that vendor. And you know how incomprehensible those can be.
The OpenBB LLM can be very useful to decode the mix of bureaucratic and marketing gibberish that plagues that type of news.
But the most useful feature is the capability to ask the LLM specific information about long earning call transcripts and other financial documents.
For example, let’s ask the OpenBB LLM some specific questions about the latest AAPL earnings call:
Eventually, this capability will be extended to all the financial documents available via the OpenBB Terminal, including SEC filings like 10-Ks and 10-Qs. Something, I’m really looking forward to.
And, as the technology matures, the OpenBB Copilot, which we have mentioned earlier, will also be able to generate ad-hoc charts starting from any data appearing in the Terminal (this is already possible today, but you have to do it manually).
If OpenBB plays its cards right, its platform might well become a gold standard in financial analysis and surpass both mature players like ThinkorSwim and new entrants like Koyfin. The company has been on my Startups Worth Watching list since 2021.
Beating Bloomberg Terminal will be a much harder task, but one step at a time.