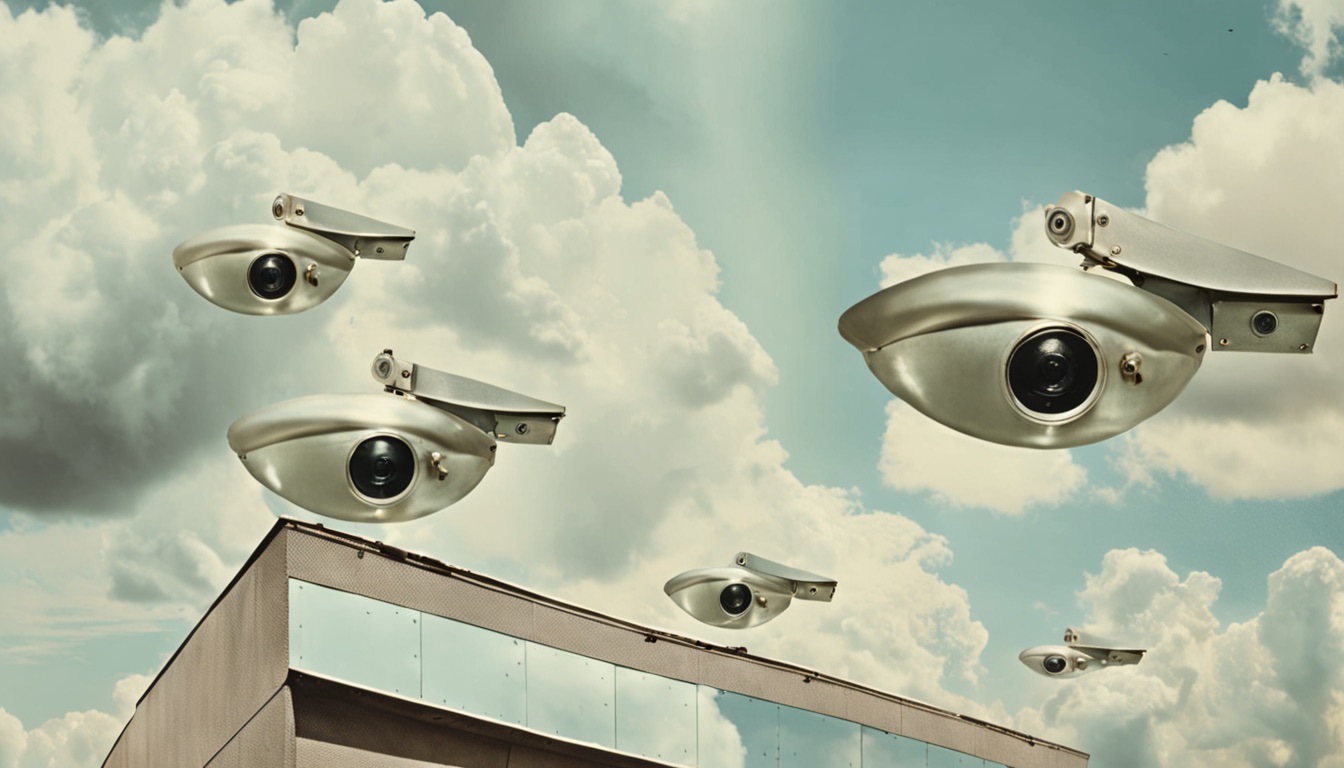
- What’s AI Doing for Companies Like Mine?
- Learn what Transport for London (TfL), Co-Op, Burger King, and the UK Advertising Standards Authority (ASA) are doing with AI.
- A Chart to Look Smart
- Promising research shows the positive impact of chatbots on 129,400 patients within England’s NHS services.
- The Tools of the Trade
- AP Workflow 8.0 for ComfyUI is out!
What we talk about here is not about what it could be, but about what is happening today.
Every organization adopting AI that is mentioned in this section is recorded in the AI Adoption Tracker.
In the Transportation industry, Transport for London (TfL) is testing AI to sport crime on the London Underground and on buses.
Matt Burgess, reporting for Wired:
Thousands of people using the London Underground had their movements, behavior, and body language watched by AI surveillance software designed to see if they were committing crimes or were in unsafe situations, new documents obtained by WIRED reveal. The machine-learning software was combined with live CCTV footage to try to detect aggressive behavior and guns or knives being brandished, as well as looking for people falling onto Tube tracks or dodging fares.
From October 2022 until the end of September 2023, Transport for London (TfL), which operates the city’s Tube and bus network, tested 11 algorithms to monitor people passing through Willesden Green Tube station, in the northwest of the city. The proof of concept trial is the first time the transport body has combined AI and live video footage to generate alerts that are sent to frontline staff. More than 44,000 alerts were issued during the test, with 19,000 being delivered to station staff in real time.
It is the first time the full details of the trial have been reported, and it follows TfL saying, in December, that it will expand its use of AI to detect fare dodging to more stations across the British capital.
In the trial at Willesden Green—a station that had 25,000 visitors per day before the Covid-19 pandemic—the AI system was set up to detect potential safety incidents to allow staff to help people in need, but it also targeted criminal and antisocial behavior.
…
The documents, which are partially redacted, also show how the AI made errors during the trial, such as flagging children who were following their parents through ticket barriers as potential fare dodgers, or not being able to tell the difference between a folding bike and a nonfolding bike. Police officers also assisted the trial by holding a machete and a gun in the view of CCTV cameras, while the station was closed, to help the system better detect weapons.
…
In a statement sent after publication of this article, Mandy McGregor, TfL’s head of policy and community safety, says the trial results are continuing to be analyzed and adds, “there was no evidence of bias” in the data collected from the trial. During the trial, McGregor says, there were no signs in place at the station that mentioned the tests of AI surveillance tools.“We are currently considering the design and scope of a second phase of the trial. No other decisions have been taken about expanding the use of this technology, either to further stations or adding capability.” McGregor says. “Any wider roll out of the technology beyond a pilot would be dependent on a full consultation with local communities and other relevant stakeholders, including experts in the field.”
…
During the London trial, algorithms trained to detect certain behaviors or movements were combined with images from the Underground station’s 20-year-old CCTV cameras—analyzing imagery every tenth of a second. When the system detected one of 11 behaviors or events identified as problematic, it would issue an alert to station staff’s iPads or a computer.
…
The categories the system tried to identify were: crowd movement, unauthorized access, safeguarding, mobility assistance, crime and antisocial behavior, person on the tracks, injured or unwell people, hazards such as litter or wet floors, unattended items, stranded customers, and fare evasion. Each has multiple subcategories.
…
“The training data is always insufficient because these things are arguably too complex and nuanced to be captured properly in data sets with the necessary nuances,” Leufer says, noting it is positive that TfL acknowledged it did not have enough training data. “I’m extremely skeptical about whether machine-learning systems can be used to reliably detect aggression in a way that isn’t simply replicating existing societal biases about what type of behavior is acceptable in public spaces.” There were a total of 66 alerts for aggressive behavior, including testing data, according to the documents WIRED received.
Like in every other use case, the secret sauce to improve the effectiveness of these algorithms will be the so-called Reinforcement Learning with Human Feedback (RLHF).
TfL needs their staff to confirm or deny the validity of the alerts generated by these image detection and classification AI models. And to do so, these AI models must be deployed in the real world.
Here in London, where I live, the Tube is incredibly safe and beloved by everyone. Kids use it to go to school, as early as in primary school, and the elderly use it to go home after a late evening theater play.
It’s by far the cleanest, most reliable, and safest public transportation system I’ve seen in my many trips around the world, after the Tokyo Metro. But it’s not perfect, especially in areas further out from the city center.
There’s an always-on, large-scale advertising campaign plastering many stations and trains focused on pushing the citizens to report harassment and suspicious behavior. I don’t have data points to evaluate the effectiveness of this campaign, but it’s easy to imagine that an always-watching AI system could be more effective than citizens fearing retaliation from violent aggressors.
If people want an even safer Tube, where women don’t feel threatened traveling alone outside peak hours, we must allow the trial of these solutions.
Let’s continue:
The most alerts were issued for people potentially avoiding paying for their journeys by jumping over or crawling under closed fare gates, pushing gates open, walking through open gates, or tailgating someone who paid. Fare dodging costs up to £130 million per year, TfL says, and there were 26,000 fare evasion alerts during the trial.
During all of the tests, images of people’s faces were blurred and data was kept for a maximum of 14 days. However, six months into the trial, the TfL decided to unblur the images of faces when people were suspected of not paying, and it kept that data for longer. It was originally planned, the documents say, for staff to respond to the fare dodging alerts. “However, due to the large number of daily alerts (in some days over 300) and the high accuracy in detections, we configured the system to auto-acknowledge the alerts,” the documents say.
…
there were almost 2,200 alerts for people going beyond yellow safety lines, 39 for people leaning over the edge of the track, and almost 2,000 alerts for people sitting on a bench for extended periods.
…
The files do not contain any analysis of how accurate the AI detection system is; however, at various points, the detection had to be adjusted. “Object detection and behavior detection are generally quite fragile and are not foolproof,” Leufer, of Access Nows, says. In one instance, the system created alerts saying people were in an unauthorized area when in reality train drivers were leaving the train. Sunlight shining onto the camera also made them less effective, the documents say.
We are undeniably moving towards a state of constant surveillance. It’s inevitable, and it will be pushed by the citizens themselves in the name of increased safety, just like I did in this newsletter.
Trying to prevent the adoption of these technologies is a lost battle that will only delay the positive outcomes they can bring.
It’s much more constructive to regulate their use and implement watchdog organizations to verify that the AI models get constantly retrained with the latest data, and that the organizations controlling these systems are held accountable for how they use them.
In the Retail industry, the supermarket chain Co-Op is implementing AI to reduce fraud at the self-checkout.
Sarah Butler, reporting for The Guardian:
The Co-op is installing in its supermarkets more than 200 secure till kiosks, locked cabinets for bottles of spirits and AI technology to monitor self-checkouts after a 44% surge in retail crime last year to about 1,000 incidents a day.
The grocery retailer, which has more than 2,400 stores across the UK, said its undercover security guards detained 3,361 individuals across its stores last year for a range of offences including burglary, abuse and harassment, amid a surge in physical assaults on its staff.
Despite spending £200m on new security measures, including additional guards and a roving undercover team targeting crime hotspots, the supermarket group suffered a 48% rise in shoplifting incidents to almost 298,000
Matt Hood, the managing director of the Co-op’s food business, said: “This is not a few opportunistic shoplifters becoming more prolific. This is organised crime and looting.”
…
Hood said the Co-op was not using facial recognition systems, unlike a number of other major chains.
…
The plan also involves the more controversial Project Pegasus under which 10 of the country’s biggest retailers, including Marks & Spencer, Boots and Primark, are handing over CCTV images to the police, to be run through databases using facial recognition technology in an effort to identify prolific or potentially dangerous individuals.
As I said in the previous story, now that we have increasingly reliable face and object recognition AI models, so cheap and efficient that can classify a face or a situation in milliseconds on a consumer computer, every organization wants to use them.
I’m not sure about the other countries, but in the UK and Italy, supermarket chains have one security guard at the entrance of the store. The effectiveness of that approach is exactly zero compared to the prospect of having your face automatically identified via CCTV and passed to the police.
But even automated facial recognition of criminals won’t be enough.
Police forces all around the world are severely understaffed and underfunded. The automation of police will be one of the most significant societal changes we’ve ever experienced. And it will be powered by artificial intelligence and robotics.
The job of the security guard in retail stores is the first one that will go.
In the Foodservice industry, Burger King is using AI in a clever marketing campaign to let customers imagine their favorite burger.
Asa Hiken, reporting for AdAge:
Burger King is taking ideas from fans for how to top a Whopper and using AI to visualize their dream recipes.
The campaign, which includes a $1 million prize, asks fans to build their burger on a microsite, with the option to add between three and eight toppings. Each topping is submitted as a text prompt, and fans are encouraged to be weird about it. Once completed, an AI companion named “Grilliam” will generate a downloadable image of the creation.
…
To further entice fans, Burger King has tied the campaign to a contest to win $1 million. In “The Million Dollar Whopper Contest,” three finalists will be selected and flown to Burger King headquarters, where they can adjust their recipes before those creations appear on menus around the country for a limited time. Finally, one of the finalists will be awarded the $1 million prize.A couple more AI gifts await those who enter the contest. Upon submission, “Grilliam” will produce a jingle personalized to the wacky Whopper, as well as a thematic background that will support the image of the burger.
…
“Grilliam” has the potential to become a PR nightmare given the freedom that consumers have to deface the Whopper, but guardrails have been established to prevent any unsavory creations. Visitors to the microsite must first agree that their customizations are edible ingredients. And even still, Grilliam might reject some ideas; Ad Age was prevented from adding peanut butter to its burger.“The Million Dollar Whopper Contest” was developed with Media.Monks, and the AI platform used to generate the images is Stable Diffusion, a person familiar with the situation told Ad Age.
Perhaps, this is just a clever marketing campaign. But what if the generated burger becomes a world best seller and Burger King decides to keep it on their menu permanently?
Would it count as AI-generated food?
In the Government sector, the UK Advertising Standards Authority (ASA) is preparing to use AI to audit 10 million ads for wrongdoing.
Kenza Bryan, reporting for Financial Times:
Advertising regulators around the world have to tackle “the scale, the volume and the pace of change” wrought by targeted and AI-generated marketing, according to Guy Parker, chief executive of the Advertising Standards Authority.
Automated tools could amplify unverified marketing buzzwords, raising some “tricky questions about where accountability lies”, said Parker in an interview with the Financial Times. Advertisers “can’t abdicate responsibility by saying, sorry, the AI did that”, he warned.
Although a decision on a promotion will ultimately be made by humans, the ASA plans to scan 10mn adverts for wrongdoing in 2024, using its own AI tool, compared to 3mn last year and just tens of thousands in 2022.
…
The ASA, which is independent of the UK government and primarily funded by an industry levy, has a data and science budget worth about five per cent of its £11mn financing for 2024.It will check that phrases like “recyclable”, “compostable” and “carbon neutral” come with sufficient context and caveats attached, while also scanning for ads that promote activities such as gambling and vaping to minors.
…
In the past year, the ASA has pivoted to an aggressive clampdown on green ads by polluters including Shell, Equinor and Lufthansa. Earlier this week, it banned emissions claims made by carmakers BMW and MG as well as claims on pollution from London’s transport authorities.In December, for example, the ASA took issue with a suggestion by Norway’s Equinor that wind farms, oil and gas, and carbon capture play a balanced role in its energy mix, when most of the company’s revenues still comes from oil and gas.
One claim that raises alarm bells is “carbon neutral”. Companies that use carbon offsets to justify this type of green claim are “kind of cheating” in the eyes of consumers, Parker said. The ASA has recently barred claims by drinks company BrewDog and shirts retailer Charles Tyrwhitt to be “carbon negative” or “carbon neutral”.
Every job, in every industry, that involves a controlling function will be automated.
You won’t believe that people would fall for it, but they do. Boy, they do.
So this is a section dedicated to making me popular.
This week, Nature published a very promising research conducted by the UK startup Limbic on 129,400 patients within England’s NHS services using a chatbot for therapy.
From the paper, titled Closing the accessibility gap to mental health treatment with a personalized self-referral chatbot:
Mental health is a global health priority as recognized by the World Health Organization1 with disorders such as anxiety and depression affecting 29% of the global population in their lifetime. The COVID-19 pandemic further exacerbated the need for more support. Besides the personal impact of these disorders, the global economic costs are estimated to be US $1 trillion annually.
The burden can be alleviated with adequate support, yet access remains limited due to structural issues such as underfunding and understaffing. Moreover, not everyone experiencing mental health problems seeks help, or they delay seeking it for years, resulting in unmet needs and inadequate support at the right time. This can be due to barriers, such as a lack of perceived need for treatment, negative attitudes and stigma, as well as structural barriers.
The first step of many mental healthcare pathways is for individuals to seek help and be referred to the appropriate healthcare service.
…
Referral to the appropriate healthcare service is pivotal, as failure to access the right support at the right time can lead to a worsening of symptoms, comorbidities and adverse outcomes, including hospitalization or suicide.
…
In the UK, the National Health Service (NHS) Talking Therapies for Anxiety and Depression program—formerly Improving Access to Psychological Therapies (IAPT)—represents a unified system for accessing mental health treatment and predominantly relies on self-referrals.Of the 1,740,652 patients referred to NHS Talking Therapies in 2021, 72% were self-referrals (NHS Digital). However, existing solutions may not be optimized or digitally streamlined, leading to lower completion rates and limited access to services.
…
Digital technologies and artificial intelligence (AI) have been proposed as potential remedies to these challenges27–29. While we30 and others31 have found evidence that digital technologies can reduce the workload of mental healthcare staff and make services more efficient, less is known about the marginal impact of digital technologies in supporting individuals of differing demographics seeking help.
…
Therefore, we developed a personalized AI-enabled chatbot solution for self-referrals, Limbic Access, which can optimize the standard referral process by autonomously gathering patient information to inform suitability for the service and initial presenting problem. We hypothesized that this user-friendly and AI-augmented self-referral could lower the threshold for accessing mental healthcare services. First, the engagement-optimized design of a personalized self-referral chatbot can guide patients proactively through the referral process and provide personalized and empathetic responses, improving the user experience and completion rates compared to webforms.Moreover, the opportunity to tailor the questioning (based on AI) towards symptoms that are especially relevant to the patient’s problems allows them to explore their mental health problems in detail38,39 and realize their need for help while reducing stigma40. Therefore, we anticipated the AI component and the user-friendliness of the chatbot to result in an increase in total referrals to services using the personalized self-referral chatbot, as well as an increase in referrals from minority groups who face more barriers to access.
In simpler terms, this startup developed a chatbot that UK practices can embed on their websites. It collects information from potential patients in a fully automated way, storing that information in the practice’s database.
Since it’s a chatbot, the idea is that people will feel more comfortable providing the information in full, rather than leave referral forms incomplete as it often happens.
More importantly, based on the conversation with the potential patient, the large language model that powers this chatbot will identify risks like self-harm or suicide, prioritizing who must be assisted based on that risk assessment.
Since the collection of data is automatic, the practice will be able to act faster in case there’s a need for immediate intervention.
And because LLMs are amazing at translating text, the chatbot can assist those portions of the UK population that struggle with the English language.
OK. Does it work?
From the paper:
We found that the services that implemented the personalized self-referral chatbot identified a 15% increase in the total number of referrals, from 30,690 to 36,070 referrals. In contrast, matched NHS Talking Therapies services with a similar number of total referrals in the pre-implementation period that used other self-referral methods, such as webforms, identified only a 6% increase in referrals in the same time period, from 30,425 to 32,240 referrals.
That’s almost 4,000 more people helped. If you think that, beyond suicide, each one of those 4,000 extra people could do something like a mass killing of innocents, all of a sudden a 15% increase in referrals becomes a very big number.
How did people react to the interaction with the chatbot?
Perhaps surprisingly to some readers, one of the upsides for patients is that they didn’t have to reveal their secrets to another human, feeling ashamed and judged.
This is why I mentioned multiple times that other AI companies not necessarily focused on health care, like Character AI, have a huge potential for mass adoption for reasons completely different than the ones intended by the founders.
Of course, these findings are also very relevant for leaders in large enterprise organizations.
As we said many times on Synthetic Work, mental health is becoming one of the most requested benefits offered by corporations in the US. And, while it’s possible that this is just a trend, corporate workers who have a healthier inner life are more productive and more inclined to collaborate with their colleagues.
On top of this, what if therapeutic LLMs could be deployed in customer service roles for customers of a, say, a retail company?
What if a more empathetic chatbot could reduce consumer complains or even increase brand loyalty and customer satisfaction?
For the most technical and creative readers among you: earlier this week I released my AP Workflow 8.0 for ComfyUI.
The biggest change is a completely new Upscaler function, much easier to configure, and capable of generating extremely high quality upscaled images, comparable or better than the output of Magnific AI (when you are aiming for maximum resemblance, not for creativity) or Topaz Gigapixel.
Magnific-style creativity is also possible (but not identical) thanks to a new Image Enhancer function.
Here’s a couple of examples:
The full list of changes in this release:
- A completely revamped Upscaler function, capable of generating upscaled images of higher fidelity than Magnific AI (at least, in its current incarnation) or Topaz Gigapixel.
- A new Image Enhancer function, capable of adding details to uploaded, generated, or upscaled images (similar to what Magnific AI does).
- The old Inpainter function is now split in two different functions: Inpainter without Mask, which is an img2img generation, and Inpainter with Mask function, which uses the exceptional Fooocus inpaint model to generate much better inpainted and outpainted images.
- A new Colorizer function which uses the u/kijai’s DDColor node to colorize black & white pictures or recolor colored ones.
- A new Aesthetic Score Predictor function can be used to automatically choose the image of a batch that best align with the submitted prompt. The automatically selected image can then be further enhanced with other AP Workflow functions like the Hand and Face Detailers, the Object and Face Swappers, or the Upscaler.
- A new Comparer function, powered by the new, exceptionally useful Image Comparer node by u/rgthree, shows the difference between the source image and the image at the end of the AP Workflow pipeline.
- The Hand Detailer function can now be configured to use the Mesh Graphormer method and the new SD1.5 Inpaint Depth Hand ControlNet model. Mesh Graphormer struggles to process hands in non-photographic images, so it’s disabled by default.
- The AI system of choice (ChatGPT or LM Studio) powering the Prompt Enricher function is now selectable from the Controller function. You can keep both active and compare the prompts generated by OpenAI models via ChatGPT against the prompts generated by open access models served by LM Studio.
- The ControlNet + Control-LoRAs function now includes six preprocessors that can be used to further improve the effect of ControlNet models. For example, you can use the new Depth Anything model to improve the effect of ControlNet Depth model.
- The Face Detailer function now has dedicated diffusion and controlnet model loading, just like the Hand Detailer and the Object Swap functions, for increased flexiblity.
- AP Workflow now supports the new version of u/receyuki’s SD Parameter Generator and SD Prompt Saver nodes.
- The LoRA Keywords function is no more. The node is now part of the Caption Generator function (but it can be used at any time, independently, and even if the Caption Generator function is inactive).
The Caption Generator function now uses the new Moondream model instead of BLIP.
Explaining how to use ComfyUI and the AP Workflow is beyond the scope of this newsletter. But some of you have started asking for tutorials or a place where to ask questions.
Given that this workflow has passed 10,000 downloads in less than six months, and I have received multiple requests for help, I’m considering how to move forward with this project.
If you have ideas or suggestions, please let me know by replying to this email.