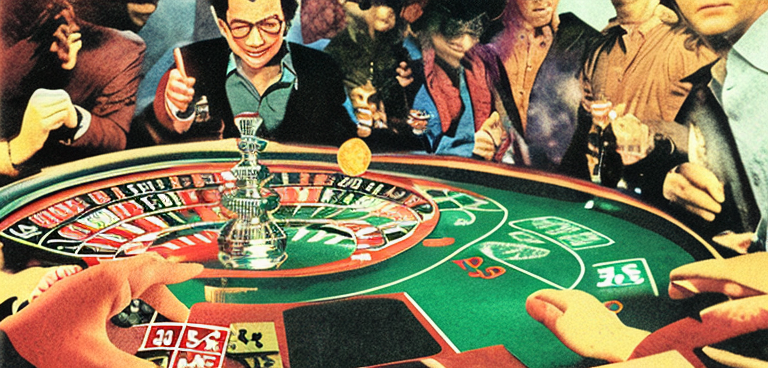
- KPMG has started using a customized version of ChatGPT that finds the right experts to pitch for a proposal within a 10,000-people database.
- The US Air Force is testing if artificial intelligence can fly F-16 fighter jets.
- Flutter Entertainment and Entain use AI to identify the so-called problem gambling.
- New Balance is using AI for footwear design.
- The Wildlife Conservation Society is using AI to design routes with the highest chances of finding poacher traps for park rangers in Cambodia.
- In the Prompting section, we make a breakthrough as we discover how AI can help solve the You don’t know what you don’t know problem.
Question. Are you happy to receive both the Free Edition and the Splendid Edition on Fridays?
If not, what day would you prefer to receive the Splendid Edition to give you a better chance to read it?
Reply to this email with the day. That’s it.
As usual, zero friction. I know you are polite and would start with “Hi.”
Thank you
Alessandro
What we talk about here is not about what it could be, but about what is happening today.
Every organization adopting AI that is mentioned in this section is recorded in the AI Adoption Tracker.
In the Professional Services industry, KPMG has started using a customized version of ChatGPT that finds the right experts to pitch for a proposal within a 10,000-people database.
Edmund Tadros, reporting for The Australian Financial Review:
The KymChat tool can securely acess internal data to quickly find experts within a 10,000-strong consulting team for use in proposals.
“The first-use case is finding people in the business,” said John Munnelly, KPMG’s chief digital officer.
…
KPMG’s KymChat, which has been created by Microsoft, is a private version of the popular ChatGPT and uses the new and improved GPT-4 language model to generate responses to user prompts.The tool can access KPMG’s internal database of partner and staff resumes and, depending on rank of the person, certain internal financial data about the firm, Mr Munnelly said. More databases will be added over time.
The data entered into the tool are kept within KPMG’s servers locally, although KymChat does access an overseas-based supercomputer to process queries.
The new chatbot comes after the firm initially blocked access to ChatGPT on its network. In mid-February, the firm unblocked access but warned partners and staff not to enter sensitive data into the tool.
The use case is pretty bland here, and it’s not clear what’s the advantage over an enterprise search approach like the one that Google offered for years. But it’s not what matters.
What matters is that this is a confirmation that GPT-4 has been designed for regulated, or at least data-sensitive, enterprise use. There is access to on-premises data (thanks to the Retrieval plugin that most users with an OpenIA GPT Plus subscription should have had access to this week). There is a mechanism to keep the sensitive data shared with the user prompt on-site. And there is a distribution model supported by Microsoft.
Remember this the next time you read somebody online saying that companies are concerned about data privacy. Apparently, if you pay, you can have a privacy-conscious GPT-4.
–
In the Defence industry, the US Air Force is testing if artificial intelligence can fly F-16 fighter jets.
Tom Ward, reporting for Wired:
On the morning of December 1, 222, a modified F-16 fighter jet codenamed VISTA X-62A took off from Edwards Air Force Base, roughly 60 miles north of Los Angeles. Over the course of a short test flight, the VISTA engaged in advanced fighter maneuver drills, including simulated aerial dogfights, before landing successfully back at base. While this may sound like business as usual for the US’s premier pilot training school—or like scenes lifted straight from Top Gun: Maverick—it was not a fighter pilot at the controls but, for the first time on a tactical aircraft, a sophisticated AI.
Overseen by the US Department of Defense, VISTA X-62A undertook 12 AI-led test flights between December 1 and 16, totaling more than 17 hours of autonomous flight time. The breakthrough comes as part of a drive by the United States Air Force Vanguard to develop unmanned combat aerial vehicles.
…
Prior to last year’s autonomous flight tests, the VISTA received a much-needed update in the form of a “model following algorithm” (MFA) and a “system for autonomous control of the simulation” (SACS) from Lockheed Martin’s Skunk Works. Combined with the VISTA Simulation System from defense and aerospace company Calspan Corporation, these updates facilitated an emphasis on autonomy and AI integration.
…
During testing in December, a pair of AI programs were fed into the system: the Air Force Research Laboratory’s Autonomous Air Combat Operations (AACO) and the Defense Advanced Research Projects Agency’s (DARPA) Air Combat Evolution (ACE). AACO’s AI agents focused on combat with a single adversary beyond visual range (BVR), while ACE focused on dogfight-style manoeuvres with a closer, “visible” simulated enemy.While VISTA requires a certified pilot in the rear cockpit as backup, during test flights, an engineer trained in the AI systems manned the front cockpit to deal with any technical issues that arose. In the end, these issues were minor
…
The Department of Defense stresses that AACO and ACE are designed to supplement human pilots, not replace them. In some instances, AI copilot systems could act as a support mechanism for pilots in active combat. With AACO and ACE capable of parsing millions of data inputs per second, and having the ability to take control of the plane at critical junctures, this could be vital in life-or-death situations. For more routine missions that do not require human input, flights could be entirely autonomous, with the nose-section of planes being swapped out when a cockpit is not required for a human pilot.“We’re not trying to replace pilots, we’re trying to augment them, give them an extra tool,” Cotting says. He draws the analogy of soldiers of bygone campaigns riding into battle on horses. “The horse and the human had to work together,” he says. “The horse can run the trail really well, so the rider doesn’t have to worry about going from point A to B. His brain can be freed up to think bigger thoughts.” For example, Cotting says, a first lieutenant with 100 hours of experience in the cockpit could artificially gain the same edge as a much higher-ranking officer with 1,000 hours of flight experience, thanks to AI augmentation.
This is not the first time that we hear this concept: AI speeds the development of a certain capability in human beings so that a notice can reach the same performance of a veteran in a very short amount of time.
We saw this in Issue #10 – The Memory of an Elephant, when a Fortune company gave its customer service reps generative AI to help them answer customers’ calls.
Let’s finish the Wired article:
This past December, trial flighs for ACE and ACCO were often completed within hours of each other, with engineers switching autonomy algorithms onboard the VISTA in minutes, without safety or performance issues, according to Cotting. In one instance, Cotting describes uploading new AI at 7:30 am and the plane being ready to test by 10 am.
“Once you get through the process of connecting an AI to a supersonic fighter, the resulting maneuvering is endlessly fascinating,” says Gray. “We have seen things that make sense, and completely surprising things that make no sense at all. Thanks to our safety systems, programmers are changing their models overnight, and we’re engaging them the next morning. This is unheard of in flight control system development, much less experimentation with unpredictable AI agents.”
See? Like we are trying to figure out the best prompt to generate that press announcement or pictures for a Powerpoint presentation, the US Air Force is trying to figure out the best prompt to kill people.
And we need to try and try and try to get better at prompting.
–
In the Gambling industry, Flutter Entertainment and Entain use AI to identify the so-called problem gambling.
Bradford Pearson, reporting for The New York Times:
Isn’t a problem gambler exactly what a casino wants financially? In short, Mr. Feldman said: no. Even putting aside regulatory issues — gambling operators can be fined or lose their licenses if they fail to monitor problem gambling and act when necessary — it is, counterintuitively, not in their best financial interest.
…
Mindway AI, a company that grew out of Aarhus University, does exactly what Mr. Feldman was skeptical of: It predicts future problem gambling. Built using research at Aarhus University by its founder, Kim Mouridsen, the company uses psychologists to train A.I. algorithms in identifying behaviors associated with problem gambling.One significant challenge is that there is no sole indicator of whether someone is a problem gambler, said Rasmus Kjærgaard, Mindway’s chief executive. And at most casinos, human detection of problem gambling focuses on just a few factors — mostly money spent and time played. Mindway’s system takes into account 14 different risks. Those include money and time but also canceled bank withdrawals, shifts in the time of day the player is playing and erratic changes of wagers. Each factor is given a score from 1 to 100, and the A.I. then builds out a risk assessment of each player, improving itself with each hand of poker or spin of the roulette wheel. Players are scored from green (you’re doing fine) to blood red (immediately step away from the game).
…In order to tailor the algorithm to a new casino or online operator, Mindway hands over its data to a group of experts and psychologists trained in identifying such behavior. (The company said they were independent, paid consultants.) They assess each client’s customers and use that model as a sort of baseline. The algorithm then replicates its diagnosis to the full customer database.
“As soon as a player profile or player behavior goes from green to yellow and to the other steps as well, we are able to do something about it,” Mr. Kjærgaard said. The value in the program isn’t necessarily just identifying those blood-red problem gamblers; by monitoring the jumps along Mindway’s color spectrum, it predicts and catches players as their play devolves. Currently, he said, casinos and online operators focus their attention on the blood-red gamblers; with Mindway they can identify the players before they ever reach that point.
…
How to actually communicate that information — and what to tell the gambler — is an ongoing debate. Some online-gaming companies use pop-up messaging; others use texts or emails. Mr. Kjærgaard hopes that clients take his data and, depending on the level of risk, reach out to the player directly by phone; the specificity of the data, he said, helps personalize such calls.Since starting in 2018, Mindway has contracted its services to seven Danish operators, two each in Germany and the Netherlands, one global operator and a U.S. sports-gambling operator, Mr. Kjærgaard said. The online gambling giants Flutter Entertainment and Entain have both partnered with Mindway as well, according to the companies’ annual reports.
…
Casino operators there use hidden cameras and facial-recognition technology to track gamblers’ betting behavior, as well as poker chips enabled with radio-frequency-identification technology and sensors on baccarat tables. This data then heads to a central database where a player’s performance is tracked and monitored for interplayer collusion.This, Mr. Kjærgaard said, is the future: The financial incentives will drive success. “Smart tables” and efforts to address money laundering and financial regulations may eventually provide the data that will supercharge the application of A.I. to in-person gambling.
This is when I tell you that, if this works, it will become yet another proof that we must surveil people with cameras everywhere. It’s for your own good, problem walker.
–
In the Footware & Apparel industry, New Balance is using AI for footware design.
In an interview to promote the upcoming Computational Design symposium in New York City, Onur Yuce Gun, Director of Computational Design at New Balance says:
My initial responsibility involed demonstrating how to integrate advanced computational design techniques into New Balance’s design and manufacturing workflows. Despite starting from scratch as a footwear designer, I persisted through numerous rounds of explaining “what is computational design?”–but don’t get me wrong, this was a two-way learning process. I started from zero as a footwear designer, so there was a steep learning curve ahead of me as well.
In the R&D process of our 3D-printing platform, TripleCell, I played a pivotal role. Starting from scratch, we were able to release two limited-production shoes in 2019. I personally designed the forefoot midsole component of the Fuelcell Echo Triple.
…
I believe that the use of AI for 3D modeling will face several challenges, particularly in terms of model fidelity and detailing. While I can envision some feasible applications of AI diffusion models in conjunction with current implicit modeling tools, I expect that AI will initially succeed in creating only basic forms, potentially ones that are structurally and materially homogeneous.
…
As the technology progresses, I anticipate that it will evolve to include gradients in the designs. However, truly finished designs always require heterogeneous solutions that incorporate a “human touch.” Increasing the resolution of a voxelized AI diffusion model may be useful in addressing these types of solutions, but the application will be limited based on the scale being dealt with.
…
We used SGANs to generate a full prototype using machine learning and 3D printing over three years ago, and while we were excited about it, we didn’t think it was enough to create a buzz around. Even back then, there wasn’t much to hype about. I simply referred to the SGAN-generated latent space videos as “Moodboard++,” where instead of pinning multiple images on a board, you allow those images to be blended using machine learning algorithms.
…
What really excited me was taking those latent space images and using them to suggest new tectonic forms. This is a way to unfold visual exploration, computational thinking and meandering.
We know that Onur uses the AI-first video editor Runway as part of his workflow, so, perhaps that’s how he creates New Balance moodboards++ these days.
–
In the Conservation industry, the Wildlife Conservation Society is using AI to design routes with the highest chances of finding poacher traps for park rangers in Cambodia.
Brian Kenny interviews Professor Brian Trelstad for Harvard Business Review:
Jonathan Palmer, who works for he Wildlife Conservation Society, otherwise known as the Bronx Zoo, and one of the principle architects of SMART, Would you add a new AI ML tool on top of a pretty decent data analytics tool that has helped park rangers manage and map their roots for patrol in conservation areas? So, do you add this new widget? Does it help? What are some of the challenges of getting it into the field? What are some of the ethical questions that you might have about increasing the chance of catching or confronting poachers if you were a ranger?
…
The SMART Coalition is a group of nine international conservation organizations that have stepped into the breach and have recognized that across the world there’s far too few rangers trying to prevent poaching from happening. And so SMART was formed as a way to use better geospatial mapping tools to enable more efficient deployment of rangers using historical data. What has happened in the past? Where have we found poachers? Where have the animals been? So, what PAWS, the Protection Assistant for Wildlife Security, is trying to do is use artificial intelligence to predict where poachers are going to be using topography, weather, road networks and historical incidents. So it’s trying to look forward. The insight of this, in fact, came from Professor Tambe’s work with port security where he has worked with the Coast Guard on identifying threats to security of ports.
…
So, SMART developed an application that extends into handhelds and GPS devices and asks for, with a pretty rigorous five-day training, to get park rangers to make note of where traps were found, where poached animals were found, where they spotted activities of poaching and log that in. And so using a handheld in the field, SMART in a fairly light touch way enables for that data to be collected as part of the routine nature of park patrolling. That data is then managed by somebody at a central place who’s been trained to look at the data and then say, “Okay, next week here’s where we think we should be patrolling, given what we’ve seen historically.” And it’s being used in over 1000 sites in 70 countries right now and has been demonstrated to reduce incidents of poaching in the sites it’s been used.
…
So, what they’re looking to do is create a shared application that takes topographical, or weather, or other economic data from the region, so Srepok in Cambodia, and feed that into computational software that will do analysis of all those different data points and provide the rangers with amplified assessment of the park, not for specific route recommendations like SMART does, but for, “Where are the hotspots? Where do we think that poaching is most likely to happen?” That translates for the park rangers into a new map of the park, which they can then decide, “Should we in fact patrol in these new hotspots.”
…
the ethical questions that come up really center around both the experience of the rangers and the poachers. And so on the one hand, the rangers want to try and deter poachers and want to try and prevent poaching, but they’re not that interested in direct conflict with the poachers themselves. There have been a handful of examples of rangers being shot and killed around the world by poachers. And so the question is whether or not the route mapping of SMART is a good enough way to make sure that you’re getting the right number of traps out of the park as quickly as you can with limited manpower. The fear with the PAWS tool, if you’re going to be going into hotspots, are you increasing the chance of potential conflict, potential armed conflict?
While the Conservation industry is not as lucrative as the Legal industry, this is a fascinating problem to approach with AI.
And, for once, it’s not about generative AI. I know you are happy about that.
But don’t worry, I’ll turn this story into a story about generative AI in a second.
Don’t believe me? Here:
All the people that will lose their jobs because of generative AI can go and work as park rangers. Win win.
There. Told you I could do it.
Before you start reading this section, it's mandatory that you roll your eyes at the word "engineering" in "prompt engineering".
The technique I want to discuss today doesn’t come from an academic paper, and I’m not even sure it qualifies as a technique per se. I suppose it’s more of a methodical approach to prompting, but it’s possibly the most valuable tool I’ve unlocked so far in my interactions with AI.
I got really excited when I discovered this method because I believe it can unlock a quality of work that is very hard to obtain otherwise.
You are all familiar with the expression: “You don’t know what you don’t know.”
That comes up often when it’s time to ponder business decisions, define strategies, perform a new task that we are not already experts about, etc.
But what if generative AI would allow us to find out what we don’t know?
Remember that, when you write a prompt, you are fundamentally pushing the AI to chart a route across this vast sea of information it has learned technically called “latent space.”
Now, latent space is a fancy way to say “I learned a gazillion things on the internet and compressed all of them into a file that is 2GB, 1TB, 10PT big.”
In other words, there’s a lot of knowledge trapped inside that latent space, including the things that we don’t know we don’t know.
But the AI knows them.
And so, what happens if we ask GPT-4 to tell us what we don’t know?
Let’s say that we need to define the product strategy for a new product. A frequently-seen use case in this section of Synthetic Work.
If I ask for some help to GPT-4, I get a fairly canonical answer that tells me what to put in a document, but I get no indication of how to arrive at that content or why it matters:
You either know very well what you are doing, or you don’t. And if you don’t this remains a challenging task, even if the AI is putting you on the right track.
In previous Splendid Editions, we saw that we can Ask for a Reason for the answers we get, and that further helps, but if we want to go deeper in understanding why we are doing what you are doing, we need to ask the AI what we don’t know:
BAAAAMMMMM!!!!
I have done business and product strategy (formally) for the last decade and this is unbelievably good.
From here, you can interrogate the AI as much as you want to fully understand these theories (and receive examples for each of them) until your newly acquired knowledge properly informs how you write your product strategy.
This approach is equally good for novices and for experts because there’s always the risk that we don’t know that don’t know something.
I’m calling this approach Get To Know What You Don’t Know.
Of course, it would be easier if we’d simply ask the AI to help with the product strategy and it would give back an answer that incorporates all these things I don’t know. But:
- The AI doesn’t do that unless we explicitly ask for it in our prompt. And we cannot explicitly ask for it in our prompt because we don’t know what we don’t know.
- If some business theories are overlapping or contradicting each other, like in this case, the AI wouldn’t know (yet) which one to apply. We could help the AI make a decision by using another technique we called Ask For Follow-up Questions, but we won’t, because we don’t know that there’s a need for it.
- We would learn nothing in the process.
Let’s close with something ambitious.
Over last weekend, OpenAI gave me access to the GPT-4 Web Browsing variant of their model, which just entered its beta phase.
If you pay the GPT Plus subscription, you get access to this one and the plug-in system.
So, what happens if we ask the AI what we don’t know with a temporal reference? What if we want to know what we don’t know about the latest business theories, instead of the most established?
As you can see the result is worse than mediocre. These are not business theories and certainly not the latest and greatest that academic research has to offer.
The reason for this disappointing result is that GPT-4 Web Browsing went looking in really questionable places to answer my question.
(Forbes Tech Council articles, if you must know)
Can we do better with a more precise prompt?
This time, the AI decided to wander on the Harvard Business School website, which is better than Forbes, but not what I wanted. More importantly, it completely lost its focus on product strategy.
Nonetheless, it will get better at this if somebody makes a GPT-4 plugin that enables access to research portals like arXiv (maybe there’s one already. I don’t have access yet to verify). And that’s something to look forward to.