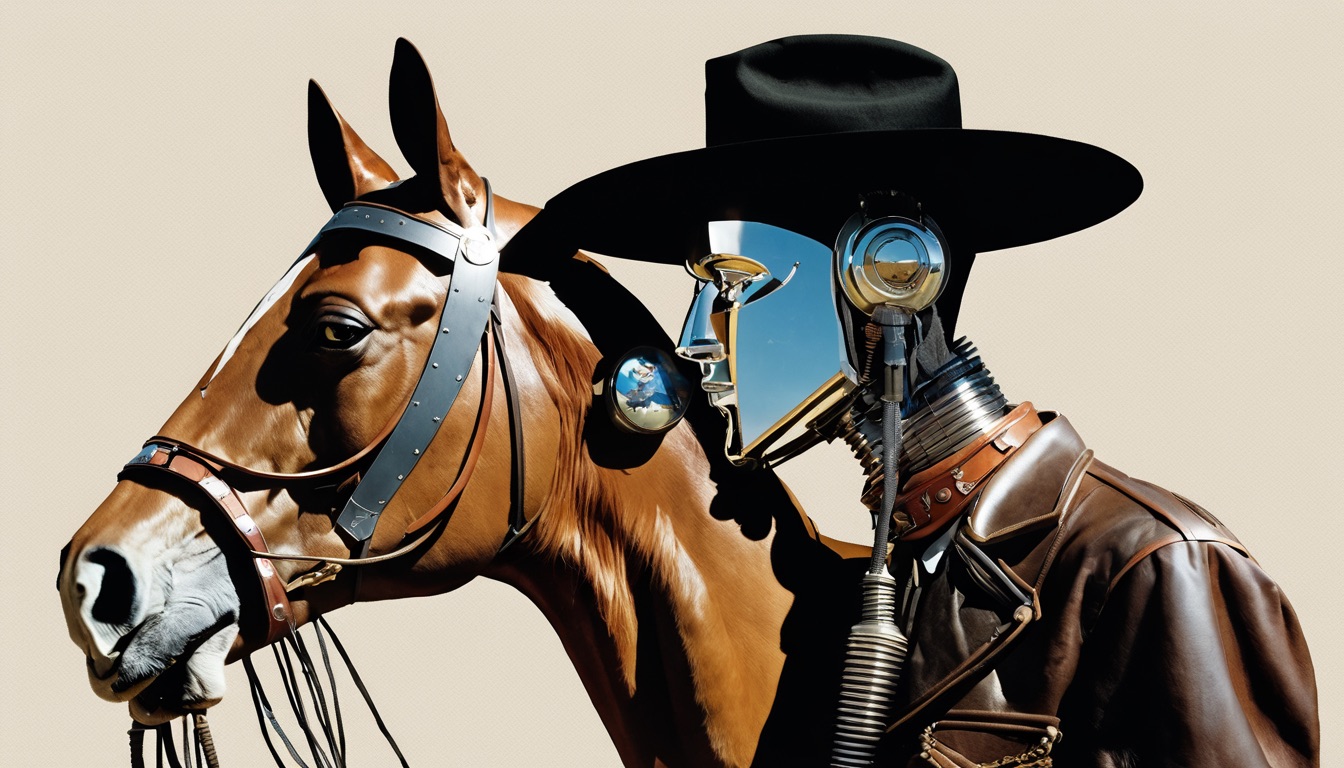
- Intro
- If you were one of the most powerful tech companies in the world, and you were given the possibility to whisper in the ears of your government, could you resist the temptation of taking advantage of it?
- What’s AI Doing for Companies Like Mine?
- Learn what Propak, JLL, and Activision are doing with AI.
- A Chart to Look Smart
- The better the AI, the less recruiters pay attention to job applications.
- Prompting
- Let’s take a look at a new technique to generate summaries dense with information with large language models. Does it really work?
- The Tools of the Trade
- Is lipsynched video translation ready for industrial applications?
Unusual for an intro, I’ll quote a new article written by Todd Feathers for Wired, focused on the sprawling adoption of ChatGPT across US government agencies:
The United States Environmental Protection Agency blocked its employees from accessing ChatGPT while the US State Department staff in Guinea used it to draft speeches and social media posts.
…
Maine banned its executive branch employees from using generative artificial intelligence for the rest of the year out of concern for the state’s cybersecurity. In nearby Vermont, government workers are using it to learn new programming languages and write internal-facing code, according to Josiah Raiche, the state’s director of artificial intelligence.
…
The city of San Jose, California, wrote 23 pages of guidelines on generative AI and requires municipal employees to fill out a form every time they use a tool like ChatGPT, Bard, or Midjourney.
…
“We’re more about what you can do, not what you can’t do,” says Sybil Gurney, Alameda County’s assistant chief information officer. County staff are “doing a lot of their written work using ChatGPT,” Gurney adds, and have used Salesforce’s Einstein GPT to simulate users for IT system tests.
…
The stakes for government employees were illustrated last month when an assistant superintendent in Mason City, Iowa, was thrown into the national spotlight for using ChatGPT as an initial step in determining which books should be removed from the district’s libraries because they contained descriptions of sex acts.
…
Seattle employees have considered using generative AI to summarize lengthy investigative reports from the city’s Office of Police Accountability. Those reports can contain information that’s public but still sensitive.
…
Staff at the Maricopa County Superior Court in Arizona use generative AI tools to write internal code and generate document templates. They haven’t yet used it for public-facing communications but believe it has potential to make legal documents more readable for non-lawyers, says Aaron Judy, the court’s chief of innovation and AI. Staff could theoretically input public information about a court case into a generative AI tool to create a press release without violating any court policies, but, he says, “they would probably be nervous.”
Why did it matter so much to be featured in the intro?
Because it dawned on me that, little by little, our policies and procedures could be re-written by generative AI.
Governments can’t compete against the private sector for talent. Not only because they can’t pay as much, but also because no innovator would want to work for a bureaucracy.
Large language models are the ultimate solution to these problems: cheap labor that can be deployed at scale, innovating a bureaucracy without the soul-crushing part of working for one.
The only problem is that, as we mentioned endless times on Synthetic Work, large language models are biased and can be steered by their creators in subtle ways to, in turn, steer even more subtly the people who interact with them.
I described how this is already happening to all of us in Issue #16 – Discover Your True Vocation With AI: The Dog Walker.
Said in another way: if you were one of the most powerful tech companies in the world, and you were given the possibility to whisper in the ears of your government, could you resist the temptation of taking advantage of it?
Alessandro
What we talk about here is not about what it could be, but about what is happening today.
Every organization adopting AI that is mentioned in this section is recorded in the AI Adoption Tracker.
In the Logistic industry, Propak is using AI to discover or anticipate, and address, safety risks in their warehouses.
Richard Vanderford, reporting for the Wall Street Journal:
“The promise of AI here is that you can actually just really up-level, by an order of magnitude, to the amount of data and the quality of data that decision makers have when they’re thinking about how they predict where the next injury is going to happen,” said Josh Butler, founder and chief executive of CompScience, whose company offers AI-driven technology that feeds into a business’s existing surveillance system.
Though a person or even a team would be hard-pressed to review all the surveillance footage produced by a typical jobsite, CompScience’s AI can sift through hours of footage to find potential safety problems.
…
Logistics company Propak uses CompScience, which can catalog near misses between forklift drivers and other employees, to watch over a 100,000-square-foot warehouse in Mira Loma, Calif. Company officials determined they had far more near misses than they thought, even though employees were supposed to come forward to report potential accidents, said Michelle McCurry, Propak’s vice president of risk management.“We wouldn’t have known that it was happening at the frequency that it was without having this type of data,” McCurry said. She said the company learned, for example, that the number of certain types of incidents went up when a particular manager went out of town.
Propak saw incidents fall sharply after it made some modifications such as providing floor markings and mirrors in problem areas, McCurry said. The company also redesigned a procedure for workers sorting produce in crates after the software warned that the ergonomics of the intense bending-and-lifting task could lead to injuries.
“We were able to actually implement change before any negative outcomes occurred,” she said.
The AI Adoption Tracker, which is now very close to tracking 100 companies across industries, gives us a unique perspective on how AI is being implemented in the real world. Sometimes, I’m really surprised by how inventive its applications can be. This is one of those times.
In the Real Estate industry, JLL is using AI to lower the carbon footprint of the buildings it manages.
Dieter Holger, reporting for The Wall Street Journal:
Keeping our buildings running contributed roughly 26% of global energy-related greenhouse-gas emissions in 2022, according to the International Energy Agency. For the world to reach net-zero emissions by 2050, the agency says the energy that these buildings consume per square meter (around 11 square feet) needs to decline by around 35% by 2030.
Developers and construction companies have pursued more-efficient energy use in buildings over the past couple of decades. Leadership in Energy and Environmental Design, or LEED, certifications are given to buildings that meet standards that conserve energy, water, waste and other environmental goals.
…
JLL, which manages billions of square feet of commercial real estate around the world, has been making a string of investments to bring AI systems to companies looking to cut their emissions. The business case: Eco-friendly buildings charge higher rents and are on the market for less time. JLL says it expects 56% of organizations to pay a premium for sustainable spaces by 2025.
…
JLL’s investments include in Turntide, a company based in Sunnyvale, Calif. that installs electric motors coupled with small computers which learn from patterns to more precisely control heating and cooling, and Envio Systems, a Berlin-based company that develops sensors to track a building’s use, occupancy and other factors to adjust lighting, cooling and similar energy-related activities.“Do I need to keep the lights on? Do I need to turn off the air conditioning on floor three because the entire company is working from home this week?,” Ravichandar said. “If you have a system, it is relentless and constantly processing this information.”
Generally, AI building systems learn from historical patterns and the daily habits of occupants to predict and power things on and off. For instance, software and hardware that automatically manages lights, heating and cooling can help buildings cut 20% or more of their yearly energy use.
…
AI has big potential to cut the emissions of buildings, but it is only as good as the data it learns from. Only 10% to 15% of buildings have the equipment or systems in place to gather the data needed to support AI, said Thomas Kiessling, chief technology officer of Siemens Smart Infrastructure. “AI in buildings works if you have the data,” he said. “Bad data means you can’t do any kind of schedules, rules or more sophisticated use cases around artificial intelligence. You have to have the data.”
We’ll measure everything. We’ll optimize everything. Until the only thing left to measure and optimize is us.
Thankfully, I already have another project for that last journey: H+.
In the Gaming industry, Activision is using AI to moderate voice chats in Call of Duty.
Monica Chin, reporting for The Verge:
First-person shooters like Call of Duty are somewhat infamous for the toxicity of their lobbies and voice chats. Surveys have dubbed the franchise’s fan base the most negative in all of gaming; a feud between two players once resulted in the summoning of a SWAT team. Activision has been trying to crack down on this behavior for years, and part of the solution might involve artificial intelligence.
Activision has partnered with a company called Modulate to bring “in-game voice chat moderation” to their titles. The new moderation system, using an AI technology called ToxMod, will work to identify behaviors like hate speech, discrimination, and harassment in real time.
ToxMod’s initial beta rollout in North America begins today. It’s active within Call of Duty: Modern Warfare II and Call of Duty: Warzone. A “full worldwide release” (it does not include Asia, the press release notes) will follow on November 10th with the release of Call of Duty: Modern Warfare III, this year’s new entry in the franchise.
You won’t believe that people would fall for it, but they do. Boy, they do.
So this is a section dedicated to making me popular.
This week, we look at a research about the negative impact of AI on hiring practices: Falling asleep at the wheel: Human/AI collaboration in a field experiment on HR recruiters.
From the abstract:
As AI quality increases, humans have fewer incentives to exert effort and remain attentive, allowing the AI to substitute, rather than augment their performance. Thus, high performing algorithms may do worse than lower-performing ones in maximizing combined output.
I then test these predictions using a pre-registered field experiment where I hired 181 professional recruiters to review 44 resumes. I selected a random subset of screeners to receive algorithmic recommendations about job candidates, and randomly varied the quality of the AI predictions they received.
I found that subjects with higher quality AI were less accurate in their assessments of job applications than subjects with lower quality AI.
On average, recruiters receiving lower quality AI exerted more effort and spent more time evaluating the resumes, and were less likely to automatically select the AI-recommended candidate. The recruiters collaborating with low-quality AI learned to interact better with their assigned AI and improved their performance. Crucially, these effects were driven by more experienced recruiters.
As someone who viscerally dislikes algorithmic hiring, I’m not surprised. Not because I think an AI model cannot evaluate resumes as good as or better than a human. Keep in mind that this research was published two years ago, before the advent of ultra-accurate models like GPT-4 and Claude.
I viscerally dislike it because, for the AI model to do its job, people get reduced to a set of dimensions that must fit one box or another.
Those that don’t fit a box, usually the ones capable of making a true difference in a company if given the latitude and resources to execute, are outright discarded.
Moreover, as AI models become more capable than humans at specific tasks, the only candidates that might be worth hiring are the ones that are not deeply specialized, but gifted with creative thinking. Those are the individuals that will be able to put AI to work to generate new revenue streams.
And so, AI for hiring, at least in the way it’s implemented today, is only speeding up the convergence of a company towards mediocrity. Human recruiters induced to sleep at the wheel, due to an ever-increasing reliance on AI models, will further accelerate the process.
Before you start reading this section, it's mandatory that you roll your eyes at the word "engineering" in "prompt engineering".
Earlier this week, AI researchers disclosed a new technique to obtain summaries from large language models that carry significantly more information within the same number of words.
They asked GPT-4 to generate increasingly dense summaries of a corpus of news, and then asked human evaluators to rate the quality of the summaries based on five metrics: informative, quality, coherence, attributable, overall preference.
They discovered that people prefer summaries dense of information. Specifically: with more information than the summaries generated by a vanilla GPT-4 prompt, but with slightly less information than the summaries generated by a human being.
So, the optimization here was not focused on achieving exactly the same performance of a human, but on making the users happy.
As I always do with the techniques that end up referenced in the How to Prompt section of Synthetic Work, I’m not going to confuse you with the names that AI researchers gave to the technique. One thing AI researchers should really stop doing is naming things.
Instead, we are going to call this new technique Less is More.
Another challenge with this technique is that the prompt is impossibly hard to remember, so be sure to go back to the How to Prompt section for a refresher.
Less is More
To see if this new technique works well, let’s compare its prompt to the prompt I use to generate the summaries for the Breaking AI News section of Synthetic Work. That is a fairly vanilla GPT-4 prompt, with the only difference that it’s focused on creating single-paragraph summaries:
Create a one-paragraph summary of the following text:
First, let’s source a random article that will serve as a basis for this: Meta Is Developing a New, More Powerful AI System as Technology Race Escalates, by the Wall Street Journal.
The parent of Facebook and Instagram is working on a new artificial-intelligence system intended to be as powerful as the most advanced model offered by OpenAI, the Microsoft-backed startup that created ChatGPT, according to people familiar with the matter. Meta aims for its new AI model, which it hopes to be ready next year, to be several times more powerful than the one it released just two months ago, dubbed Llama 2.
The planned system, details of which could still change, would help other companies to build services that produce sophisticated text, analysis and other output. It is the work of a group formed early this year by Meta Chief Executive Mark Zuckerberg to accelerate development of so-called generative AI tools that can produce humanlike expressions. Meta expects to start training the new AI system, known as a large language model, in early 2024, some of the people said.
Plans for the new model, which haven’t previously been reported, are part of Zuckerberg’s effort to assert Meta as a major force in the AI world after it fell behind rivals. Competition in the area has sharply intensified this year, spawning divergent views on everything from which business models are best to how the technology should be regulated.
The company is currently building up the data centers necessary for the job and acquiring more H100s, the most advanced of the Nvidia chips used for such AI training. While Meta joined with Microsoft to make Llama 2 available on Microsoft’s cloud-computing platform Azure, it plans to train the new model on its own infrastructure, some of the people said.
Zuckerberg is pushing for the new model, like Meta’s earlier AI offerings, to be open-sourced and therefore available free for companies to build AI-powered tools.
Zuckerberg will be among a group of top tech executives attending a summit organized by Senate Majority Leader Chuck Schumer (D., N.Y.) on Wednesday to discuss how to handle AI. Sam Altman, OpenAI’s CEO, and Sundar Pichai, Google’s CEO, will also be attending.
The model under development may not close the gap with Meta’s competitors.
Meta hopes it will be roughly as capable as GPT-4, which OpenAI launched in March. GPT-4 underpins OpenAI’s moneymaking initiatives such as the recently launched ChatGPT for Business tool, and the company has been courting others to build on top of the technology as it tries to cover the enormous costs for advanced AI models. Meta’s new model also likely would come out after the expected debut of Gemini, an advanced large language model being built by Google.
Meta’s open-sourced approach has certain advantages. Zuckerberg has championed open-source AI models, which are popular for their lower cost and adaptability.
There also are potential downsides to an open-source model of the power Meta aspires to, say some legal specialists. These include increased risks around use of potentially copyright-protected information and broader access to a tool whose enhanced strength can be used to generate and spread disinformation or other bad actions.
Meta’s lawyers have raised some of these concerns as part of their review of the company’s plans.
“You can’t easily predict what the system would do or its vulnerabilities—what some open source AI systems offer is a limited degree of transparency, reusability and extensibility,” said Sarah West, a former adviser to the Federal Trade Commission who is now managing director of the AI Now Institute, a research institute that has raised concerns about big companies’ control over AI.
Large language models generally get more powerful when trained on more data. The most powerful version of the Llama 2 model that Meta announced in July was trained on 70 billion parameters, a term for the variables in an AI system that is used to measure size. OpenAI hasn’t disclosed the size of GPT-4, but it is estimated to be roughly 20 times that size, at 1.5 trillion parameters. Some AI experts say there could be other methods to achieve GPT-4’s power without necessarily approaching its size.
This is what my Breaking AI News prompt would generate as a summary:
Which is pretty good.
Let’s see what happens with the Less is More prompt:
Before we continue, let’s see what’s happening here.
The first thing to notice is that the prompt is asking for a recursive action. GPT-4 has to repeat the two steps described in the prompt for five times.
Each time, the summary should incorporate valuable, but missing information from the original text.
This approach is similar to the one we discussed when I introduced the Summarize the Conversation technique. At the end of the day, the goal is similar.
So, let’s see how well GTP-4 follows the instructions:
Notice the trick of filling the first summary with highly generic information in action. It’s an interesting technique.
Let’s see what happens in the next two iterations:
GPT-4 continues to perform well. Let’s see what happens in the final, fifth iteration:
Notice how GPT-4 has lost track of the most important thing: there will be a successor to LLaMA and it will be as powerful as GPT-4.
A person who doesn’t follow what’s happening in the world of AI, reading this summary, would assume that LLaMA 2 is not yet out and that is Meta’s hope to compete against GPT-4.
On top of that, as you can see, GPT-4 still has a few entities to incorporate.
At this point, I would declare the technique unreliable, but here’s a surprise: if we ask GPT-4 to continue, it performs a 6th loop:
This summary is even more confusing than the fifth one, so I wouldn’t go further than this.
At this point, I would conclude the experiment, but then I thought: what if we remove the following sentence from the prompt?
Remember, use the exact same number of words for each summary.
The result we get in the fifth summary is better in terms of retaining the key information, albeit less elegant:
But, again, if we push for a sixth iteration, things start to get confusing:
Overall, this technique produces unreliable results. Perhaps the researchers are onto something, but the prompt seems to need more refinement.
While you wait for good news, you could try the Less is More technique with the open access model Falcon180B, which is ready to answer your questions on the Discord server of Synthetic Work.
I’ll check your experiments over there.
Normally, I use this section of the Splendid Edition to recommend life-changing tools infused with AI, or about AI, that will make a tangible impact on your productivity and the productivity of your workforce.
Occasionally, I have to use this space to warn you against using certain tools that claim performance they cannot deliver.
Today is one of those days.
Unless you have detoxed from social media for the past week, you might have seen an avalanche of short clips made by AI enthusiasts about a company called HeyGen and their new beta service called Video Translate.
Video Translate is the holy grail of media production: an AI system that promises to take a video in any supported language and:
- Capture the accent, tone, pitch, etc. of the speaker’s voice.
- Translate the spoken text in a target language.
- Speak the translated text with the cloned voice obtained in step #1.
- Lipsync the translated speech in a target video, with no visible artifacts.
For this to happen, HeyGen and its competitors would have to coordinate an impressive number of AI models, each trained for a different task: speech recognition, voice cloning, speech synthesis, face recognition, image2image generation for the lip-syncing part, etc.
It’s an impressive pipeline that must be executed at huge speed and scale to be useful for media companies.
Should any of them succeed, we would have every video in the world automatically translated into any language of the world, opening up infinite possibilities for media companies.
Well, if you watch this demonstration on X, you might think that this is it. We are there:
Except that we are not. We are not even close.
To test if the technology is really ready for industrial applications, I cut a short clip out of the latest episode of Le Voci dell’AI, the weekly video podcast I publish in Italian for 01net.it.
Hence, the starting language is Italian, one of the supported languages in this beta version of Video Translate.
I tried three different translations: in English, as some readers of Synthetic Work wished they could understand that podcast, and in French, as 01net is a French media company.
The English video translation comes in two flavors: a version that captures the original voice of the speaker, and a version that uses a professional voice.
All versions use the AI voice technology developed by ElevenLabs, the company I used to create an audio version of Synthetic Work Issue #28 Free Edition.
So, how did it go?
Let’s start with the original clip, in Italian:
Now, let’s see what happens when I try to voice it in English with my own voice (at least according to HeyGen description):
You can immediately see the enormous problems in terms of out-of-sync lip movements and artifacts on my face.
A key challenge we previously discussed is that some languages are faster than others in terms of pronunciation. Hence, any AI model has to take into account that difference to produce a convincing result.
The English version with a stock American voice doesn’t do much better:
In fact, even worse. As you noticed, I tried to increase the resolution of the video clip, to see if it would make a difference.
Finally, the French version, which I cannot even express an opinion on because I studied French in secondary school for just three years, a million years ago:
To do this test I paid for the regular version of HeyGen. So there are no restrictions due to a free plan trial. It’s just not ready.
And it’s not just HeyGen. I spent a week researching all video dubbing with lip-syncing services on the market, and they all are proof of concepts at best, consulting jobs at worst.
This technology is amazing and HeyGen is making enormous progress, but this is not ready for prime time. Postpone exploration.