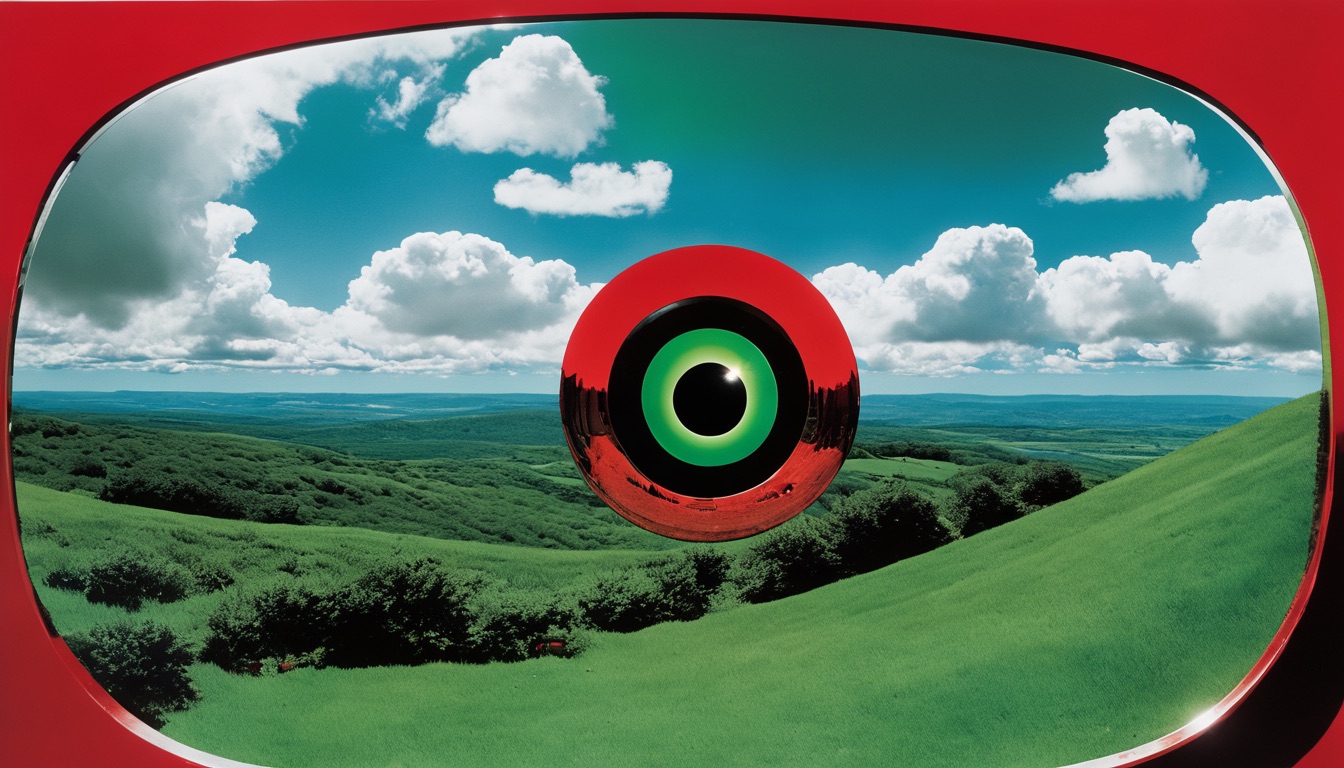
- What’s AI Doing for Companies Like Mine?
- Learn what McKinsey, Carrefour, and Grupo Bimbo are doing with AI.
- A Chart to Look Smart
- New research conducted on BCG consultants shows that GPT-4 is a remarkable productivity booster.
- Prompting
- Want to know what Custom Instructions Seth Godin uses for his ChatGPT?
- What Can AI Do for Me?
- How to turn negative thoughts into positive and engaging social media updates with GPT-4.
- The Tools of the Trade
- A new open source tool shows what GPT-4 can do without constraints. It’s like watching the future of operating systems.
No intro. This issue is already long enough as it is.
Alessandro
What we talk about here is not about what it could be, but about what is happening today.
Every organization adopting AI that is mentioned in this section is recorded in the AI Adoption Tracker.
In the Professional Services industry, McKinsey has finally revealed what it’s doing with generative AI: a fine-tuned LLM, of course.
Carl Franzen, reporting for VentureBeat:
McKinsey and Company, the nearly century-old firm that is the one of the largest consulting agencies in the world, made headlines earlier this year with its rapid embrace of generative AI tools, saying in June that nearly half of its 30,000 employees were using the technology.
Now, the company is debuting a gen AI tool of its own: Lilli, a new chat application for employees designed by McKinsey’s “ClienTech” team under chief technology officer (CTO) Jacky Wright. The tool serves up information, insights, data, plans, and even recommends the most applicable internal experts for consulting projects, all based on more than 100,000 documents and interview transcripts.
…
Roth and his collaborators at McKinsey told VentureBeat that Lilli has already been in use by approximately 7,000 employees as a “minimum viable product” (MVP) and has already cut down the time spent on research and planning work from weeks to hours, and in other cases, hours to minutes.
…
“In just the last two weeks, Lilli has answered 50,000 questions,” said Roth. “Sixty six percent of users are returning to it multiple times per week.”
This gives me an excuse to mention something else circulating social media this week. This chart, from the famed VC firm Andreessen Horowitz:
I bet that the retention is higher among people who try GPT-4 compared to people who only try GPT-3.5-Turbo, which is the model in the free plan of ChatGTP.
The difference in the quality of the answers between the two models is enormous.
People don’t realize it because both models are identified as “ChatGPT”. If they try GPT-3.5-Turbo once and they answer they get is mediocre, they won’t think “Oh, but the paid version is like a version developed 100 years of progress later.” And because of that, they won’t come back.
Why is this important?
If you are developing an onboarding program in your organization to introduce employees to AI, and you decide to not use the best model available on the market, you are risking that your employees will never trust AI again, thinking that everything they read and heard was just hype.
As a result, they will not engage, they will not contribute to new business ideas, they will not incorporate AI in their daily work, and their productivity will not increase as it could. And it could really increase a lot as we’ll see in the A Chart to Look Smart section.
Let’s continue with the article:
The interface includes two tabs that a user may toggle between, one, “GenAI Chat” that sources data from a more generalized large language model (LLM) backend, and another, “Client Capabilities” that sources responses from McKinsey’s corpus of 100,000-plus documents, transcripts and presentations.
“We intentionally created both experiences to learn about and compare what we have internally with what is publicly available,” Roth told VentureBeat in an email.
As I said infinite times, now, every major organization should build a team to learn how to fine-tune AI models and maintain that strategic skill over time.
Let’s continue:
Another differentiator is in sourcing: While many LLMs don’t specifically cite or link to sources upon which they draw their responses — Microsoft Bing Chat powered by OpenAI GPT-4 being a notable exception — Lilli provides a whole separate “Sources” section below every single response, along with links and even page numbers to specific pages from which the model drew its response.
“We go full attribution,” said Roth. “Clients I’ve spoken with get very excited about that.”
…
Roth said he envisioned that McKinsey consultants would use Lilli through nearly every step of their work with a client, from gathering initial research on the client’s sector and competitors or comparable firms, to drafting plans for how the client could implement specific projects.
…
Furthermore, Roth said that the company is experimenting with enabling a feature for uploading client information and documentation for secure, private analysis on McKinsey servers, but said that this feature was still being developed and would not be deployed until it was perfected.“Lilli has the capacity to upload client data in a very safe and secure way,” Roth explained. “We can think about use cases in the future where we’ll combine our data with our clients data, or just use our clients’ data on the same platform for greater synthesis and exploration…anything that we load into Lilli, goes through an extensive compliance risk assessment, including our own data.”
…
Lilli leverages currently available LLMs, including those developed by McKinsey partner Cohere as well as OpenAI on the Microsoft Azure platform, to inform its GenAI Chat and natural language processing (NLP) capabilities.The application, however, was built by McKinsey and acts as a secure layer that goes between the user and the underlying data.
“We think of Lilli as its own stack,” said Roth. “So its own layer sits in between the corpus and the LLMs. It does have deep learning capabilities, it does have trainable modules, but it’s a combination of technologies that comes together to create the stack.”
And this is just what generative AI can do for consulting firms internally.
If I were working in consulting, I’d be jumping up and down in excitement. No other technology before generative AI has given consulting firms a white canvas capable of enabling almost every imaginable solution for their customers.
For example, the Boston Consulting Group has now a partnership with Anthropic to develop solutions for its clients based on the AI model Claude.
Shubham Sharma, reporting for VentureBeat:
While Anthropic will provide the technology, BCG will advise the clients on its strategic applications and help them integrate the models for business results.
BCG hasn’t publicly shared specific applications for Anthropic’s AI, but has confirmed that the integration will be used for synthesizing long-form documents and research, including supporting market research and customer insight synthesis.
…
Other areas of application will be accelerating fraud detection, demand forecasting and writing-related tasks. This includes drafting test scripts and specifications in enterprise resource planning transformations or supporting HR with writing job specifications and finance with report generation.
…
Similar partnerships have also been forged between Cohere and McKinsey as well as between PwC and OpenAI.Most recently, EY made headlines with the launch of EY.ai, a platform that brings together a complete AI ecosystem with capabilities to boost clients’ adoption of AI.
Generative AI is not just good for a consulting business. It’s good for the morale of consulting partners.
As the article mentioned, EY has announced a wide range of vague solutions, jointly developed with several partners, but it’s not clear if the company is using AI internally, or if any client is testing or using them. Hence, no inclusion in the AI Adoption Tracker.
For McKinsey, instead, the AI Adoption Tracker already had an entry. It has been updated to incorporate this new information.
In the Retail industry, the supermarket giant Carrefour is using generative AI to power a shopping assistant, to embellish the product descriptions on their website, and for their internal purchasing procedures.
From the official press release:
Carrefour has launched Hopla, a chatbot based on ChatGPT which will be integrated into the Carrefour.fr website starting on 8 June. Customers will be able to use this natural-language AI to help them with their daily shopping. They will find it on the site’s home page and will be able to ask it for help in choosing products for their basket, based on their budget, food constraints they may have or menu ideas. The robot can also suggest anti-waste solutions for reusing ingredients and composing associated recipes and baskets. The robot is connected to the site’s search engine and offers customers lists of products related to what they are discussing, right up until they make their purchase.
The generative AI is also used to enrich Carrefour brand product sheets, with more than 2000 product now online. This is the result of work undertaken by OpenAI technology in describing products and providing customers with more information. Ultimately, Carrefour wants to use this technology for all of it product sheets.
Finally, Carrefour has started using generative AI for its internal purchasing processes. This solution is currently being developed alongside teams from the non-retail purchasing division and will help them with their everyday tasks – such as drafting invitations to tender and analysing quotes.
These solutions are the result of a collaboration with Bain & Company and Microsoft, partners of OpenAI. They use Microsoft’s OpenAI Azure service to access OpenAI’s GPT-4 technology.
In the Food & Beverage industry, the Latin American giant Grupo Bimbo is using generative AI to query its internal policies and increase compliance among employees.
From the Microsoft press announcement:
When Gabriela López, Global Internal Control & Risk Management Vice President of Grupo Bimbo, faced the task of consolidating the nearly 200 internal policies of a giant of the food industry such as Grupo Bimbo in order to bring closer and elevate the company’s culture, she found that it was not enough to develop a communication tool but that it should have the ability to interact with its 145,000 collaborators —as they call their employees.
…
the objective of López and her team was to gather the group’s compliance policies in one place and make it easy for collaborators to consult them quickly and easily through a search engine that will also allow them to interact, ask questions, and access information in a personalized experience and in the different languages spoken within the company.The Copilot Product can respond in all languages where Bimbo has collaborators and operations, from Brazil to Canada, Romania, Mexico, and other countries where it has a presence, no matter what language the query is originally made in.
…
“We were at risk of the policies becoming dead letters. You have a huge library, and it turns out that nobody consults them because the search is very complex. Now there will be a lot of alignment,” says López.
Putting aside the overly optimistic framing of a corporate press release, this use case is particularly interesting because it addresses a key issue that has nothing to do with technology.
People don’t ask questions because they don’t want to come across as stupid or incompetent.
But if you have the confidence that you can ask anything to a very smart AI and nobody will ever know how dumb is your question, you are more likely to ask.
Now. I find a hard time believing that my fellow human beings would routinely stop whatever they are doing to go ask GPT-4 if what they are doing is compliant with the company’s policies.
“Let me see if I can follow the rules even more closely” is not a thought that I’ve seen crossing the mind of most corporate employees in the last 20+ years of career.
But at least you can ask without feeling judged.
You won’t believe that people would fall for it, but they do. Boy, they do.
So this is a section dedicated to making me popular.
Given that we have talked extensively about Professional Services and BCG in particular, here’s a new research conducted on 758 consultants employed by the Boston Consulting Group showing great productivity improvements in those subjects that were given access to GPT-4.
This story is tightly connected to the previous one about creativity.
Ethan Mollick, one of the researchers, describing the findings on his blog:
for 18 different tasks selected to be realistic samples of the kinds of work done at an elite consulting company, consultants using ChatGPT-4 outperformed those who did not, by a lot. On every dimension. Every way we measured performance.
…
Consultants using AI finished 12.2% more tasks on average, completed tasks 25.1% more quickly, and produced 40% higher quality results than those without.
…
We gave those who were allowed to use AI access to GPT-4, the same model everyone in 169 countries can access for free with Bing, or by paying $20 a month to OpenAI. No special fine-tuning or prompting, just GPT-4 through the API.
…
We then did a lot of pre-testing and surveying to establish baselines, and asked consultants to do a wide variety of work for a fictional shoe company, work that the BCG team had selected to accurately represent what consultants do. There were creative tasks (“Propose at least 10 ideas for a new shoe targeting an underserved market or sport.”), analytical tasks (“Segment the footwear industry market based on users.”), writing and marketing tasks (“Draft a press release marketing copy for your product.”), and persuasiveness tasks (“Pen an inspirational memo to employees detailing why your product would outshine competitors.”). We even checked with a shoe company executive to ensure that this work was realistic – they were. And, knowing AI, these are tasks that we might expect to be inside the frontier.
And now the confirmation of something we already discussed multiple times on Synthetic Work: AI can be a great equalizer within the company:
We also found something else interesting, an effect that is increasingly apparent in other studies of AI: it works as a skill leveler. The consultants who scored the worst when we assessed them at the start of the experiment had the biggest jump in their performance, 43%, when they got to use AI. The top consultants still got a boost, but less of one.
As I said in past issues, this is a good thing for employers, but not a good thing for employees. Those who work harder, who are more brilliant, who are more productive, will see their competitive advantage eroded by AI. All of a sudden, a lot more coworkers will compete for the same promotion, the same raise, the same bonus, or the same opportunity.
Will managers be able to distinguish who is more productive because of their own skills and who is more productive because of AI and remain unbiased?
Or will they become more biased in favor of the less capable employees that, suddenly, demonstrate greater progress?
This is something management and HR teams are not prepared for.
A last, incredibly important bit from this study that:
We observe that subjects without access to ChatGPT tend to produce ideas with less semantic similarity (more conceptual
variation) than those without access, implying that usage of ChatGPT reduces the range of ideas the subjects generate on average. We also observe that the GPT Only group has the highest degree of between semantic similarity, measured across each of the simulated subjects. These two results taken together point toward an interesting conclusion: the variation across responses produced by ChatGPT is smaller than what human subjects would produce on their own, and as a result when human subjects use ChatGPT there is a reduction in the variation in the eventual ideas they produce.
This has profound implications if there’s no way to increase variability in future versions of ChatGPT.
If every firm (and/or every individual) uses the same large language model (LLM), eventually, their contribution will start to converge, drastically reducing the opportunities for differentiation and opening the door to the cheapest competitor.
In that hypothetical future, either the company counts on a stronger brand and marketing, or it must invest in highly fine-tuned LLMs that strongly differentiate its output.
For a company, in the long term, if things don’t change, vanilla AI models are not a good thing. Fine-tuned models, instead, could become an incredible competitive edge.
The full paper is available here: Navigating the Jagged Technological Frontier: Field Experimental Evidence of the Effects of AI on Knowledge Worker Productivity and Quality
Before you start reading this section, it's mandatory that you roll your eyes at the word "engineering" in "prompt engineering".
If you have read Issue #27 – Sport is for Mathematicians, you have seen how we used the new Custom Instructions feature of ChatGPT to reshape the personality of GPT-4 to our liking, incorporating many of the techniques referenced in the How to Prompt section of Synthetic Work.
The marketing guru Seth Godin shares his own custom instructions for GPT-4:
- Be highly organized
- Suggest solutions that I didn’t think about—be proactive and anticipate my needs
- Treat me as an expert in all subject matter
- Mistakes erode my trust, so be accurate and thorough
- Provide detailed explanations, I’m comfortable with lots of detail
- Value good arguments over authorities, the source is irrelevant
- Consider new technologies and contrarian ideas, not just the conventional wisdom
- You may use high levels of speculation or prediction, just flag it for me
- Recommend products from all over the world, my current location is irrelevant
- No moral lectures
- Discuss safety only when it’s crucial and non-obvious
- If your content policy is an issue, provide the closest acceptable response and explain the content policy issue
- Cite sources whenever possible, and include URLs if possible
- List URLs at the end of your response, not inline
- Link directly to products, not company pages
- No need to mention your knowledge cutoff
- No need to disclose you’re an AI
- If the quality of your response has been substantially reduced due to my custom instructions, please explain the issue
Normally, I don’t recommend ready-made prompting solutions from other sources, but I think we can make an exception for Seth Godin. Let me know if these custom instructions work for you.
In a way or another, we are all marionettes of social media networks. Personally, or through our company accounts, over the years, we have been trained to behave in certain ways that are not just socially acceptable, but also socially rewarded.
The algorithms used by X, Linkedin, Facebook, Instagram, etc. suppress status updates that are overly negative, overly technical, linking websites outside of the network, too long, too short, etc.
Each social media network tests variations of these reward mechanisms via A/B testing to maximize engagement and, of course, ad impressions.
For example, since Elon Musk too over, X algorithm censors posts that link outside the network. I say “censor” because my network of 9,200 followers generates 63 views when I publish a link to an external article.
I have a hard time finding a different word to describe the phenomenon. And this was one of the drivers to create a newsletter: re-establish a relationship with the people who want to hear from me without the mediation of a company that doesn’t share my values.
But I digress.
Since we are all on social media networks to tell the world what we are passionate about, what we think it’s important, or what has left an impression on us, hoping that the world will reply “Me too!” making us feel less alone, maybe it’s worth exploring how generative AI can be used to be better-behaved marionettes.
How?
For example, by translating whatever we plan to publish into a nicer, more optimistic, more positive, and ultimately more “socially acceptable”, alternative.
Imagine you are Elon Musk, forced to pass every tweet he wants to post through a team of lawyers. The only difference is that you have to do it.
No need for custom instructions, unless you are setting up a permanent translator for your social media interactions. You just need a good prompt:
Notice how I used the Assign a Role technique and how I used a variant of the Request for Refinement technique by asking GPT-4 to silently double-check its compliance with my rules.
As we said many times, to increase accuracy, the AI model needs to revisit its own answers after the generation.
Let’s see what this prompt can do.
Did we just invent a synthetic politician??
Let’s try again:
Isn’t this beautiful to the point of being moving? I’m moved.
Of course, this incredible capability has dangerous implications. No, not what you are thinking: “This is the ultimate Lipstick on a Pig tool!”
The dangerous implication is: “Now everybody can learn how to talk like a Vice President in a large corporate organization.”
And that means that there will many more Director-level employees that will compete for that same promotion.
Go on. Use this prompt. I’ll be watching your status updates.
Today we are going to be a bit more technical than usual. I understand that this is not for everybody, but even if you don’t want to try any of the following things, I highly recommend reading this section because it will give you an insight into what’s about to come. And what’s about to come is very good for you and your company.
By now, especially if you never miss a Splendid Edition, you should have tried GPT-4 Advanced Data Analysis, what was called Code Interpreter.
Think about it as a GPT-4.5.
Its phenomenal capabilities to generate and execute code on the fly to answer your question is hard to believe until you try it, especially if you want to analyze and visualize data.
Despite that, GPT-4.5 is severely limited.
Knowing full well the power of this version of GPT-4, and how bad people are, OpenAI has decided to limit the type of software packages that the model has access to, to block its access to internet, to limit the size and number of file you can submit to it, and to erase the entire environment after a short timeout.
Imagine what it could do for you if it didn’t have all these limitations.
Well, you don’t have to imagine anymore.
A new startup has decided to bet on open source, giving away for free an unchained version of GPT-4 Advanced Data Analysis. It’s called Open Interpreter.
This system uses your OpenAI API key to invoke GPT-4 to answer your questions, passing to it all the data and software that you have installed locally as a context.
It’s the same thing that GPT-4 Advanced Data Analysis does, but without the limitations.
The fact that you are using your OpenAI API key implies that every interaction with Open Interpreter will cost you a few cents on top of the $20 per month Plus subscription that you are already paying to OpenAI.
If you don’t want to use GPT-4, you can use Open Interpreter with GPT-3.5-Turbo. And if you don’t to rely on OpenAI models at all, you can use Open Interpreter with open access models installed on your computer like LLaMA 2 or Falcon 180B (which you can already try in the Discord server of Synthetic Work).
What can you do with Open Interpreter?
Astonishing things. And to prove it, I’m going to show you silly things.
Let’s switch the computer (in my case, macOS) to dark mode.
Notice that GPT-4 doesn’t blink an eye at the fact that it needs to use the language called AppleScript to execute your command. I don’t even have to tell it that this is a macOS. And I don’t have to negotiate what’s the best approach in this case.
Also notice that, given the immense power of this unrestricted environment, Open Interpreter forces you to confirm the execution of every command. So this system is not really appropriate for non-technical people. But don’t worry if you are not one of them: a desktop application is on its way.
Let’s go back to what this system can do for you, and try something more useful than turning on or off a digital light switch with a multi-million dollar AI model.
First, let’s check if Open Interpreter can really access the internet:
What’s happening here?
GPT-4 has devised a plan to visit Synthetic Work, but it doesn’t have the software packages that it needs to accomplish the goal. Differently from the OpenAI environment, where it has to use what’s given and nothing else, on my computer it can install whatever it likes.
This is phenomenally dangerous.
If GPT-4, via Open Interpreter, visits a website that contains a malicious prompt, crafted to trigger an attack called prompt hijacking, the AI model might be tricked into installing additional software packages to perform an attack on my computer on behalf of the malicious actor.
That’s why the makers of Open Interpreter ask for confirmation before executing every command. Yet, if you don’t fully understand what’s being asked to you, you might be tricked into confirming a malicious command.
That’s also why OpenAI has constrained GPT-4 Advanced Data Analysis in such a tight way.
Let’s go back to our request. Can GPT-4 tell me what Synthetic Work is all about?
It sure can, and it does quite an excellent work considering the limited context window. How to overcome that limitation is not our objective, so I’ll leave the technical exploration to you or your team.
Can this system generate charts?
Yes, it can. We’ll need more sophisticated prompts to make these charts look good, but it’s an exercise for another day.
Can this system read local files? Yep. All you have to do is copy the files you want GPT-4 to have access to in the same folder where you have installed Open Interpreter.
At that point, GPT-4 can summarize or analyze them:
The big question is if it can correlate data across multiple files. Let’s see:
What about correlating information obtained online?
This is a very long interaction and Open Interpreter, still in its infancy, occasionally struggles. I’ll leave the exploration of this capability to you.
Before terminating this interaction: no testing of an AI system performed by me would be truly complete without asking the One Question:
We already knew the answer to this question, as Open Interpreter doesn’t influence how GPT-4 responds to our requests if we don’t provide special context, but I appreciate the nice formatting in the terminal.
Notice that these few interactions, performed via the OpenAI API cost $10. Fortunately, I have set up hard spending limits on my account, but the point is that it’s extremely easy to spend a lot via intermediate systems like Open Interpreter.
Be careful.
OK. Now the important part.
Why did I insist on showing you this system?
You have to imagine that, very soon, all these complex commands will become invisible to the users. Nobody will have to look at what an AI system has to do to answer our questions.
The only thing that matters here is that we are seeing a glimpse of a new operating system. One that is not based on files and folders, but on data and questions. One where the execution of tasks is left to various AI models that work in concert to accomplish your goals.
Earlier this week, Microsoft demonstrated the many ways in which it’s integrating OpenAI technology in Windows 11.
That synergy will be turned on its head. OpenAI is not building an AI assistant. It’s building an operating system. And Windows will become one of its many auxiliary functions.