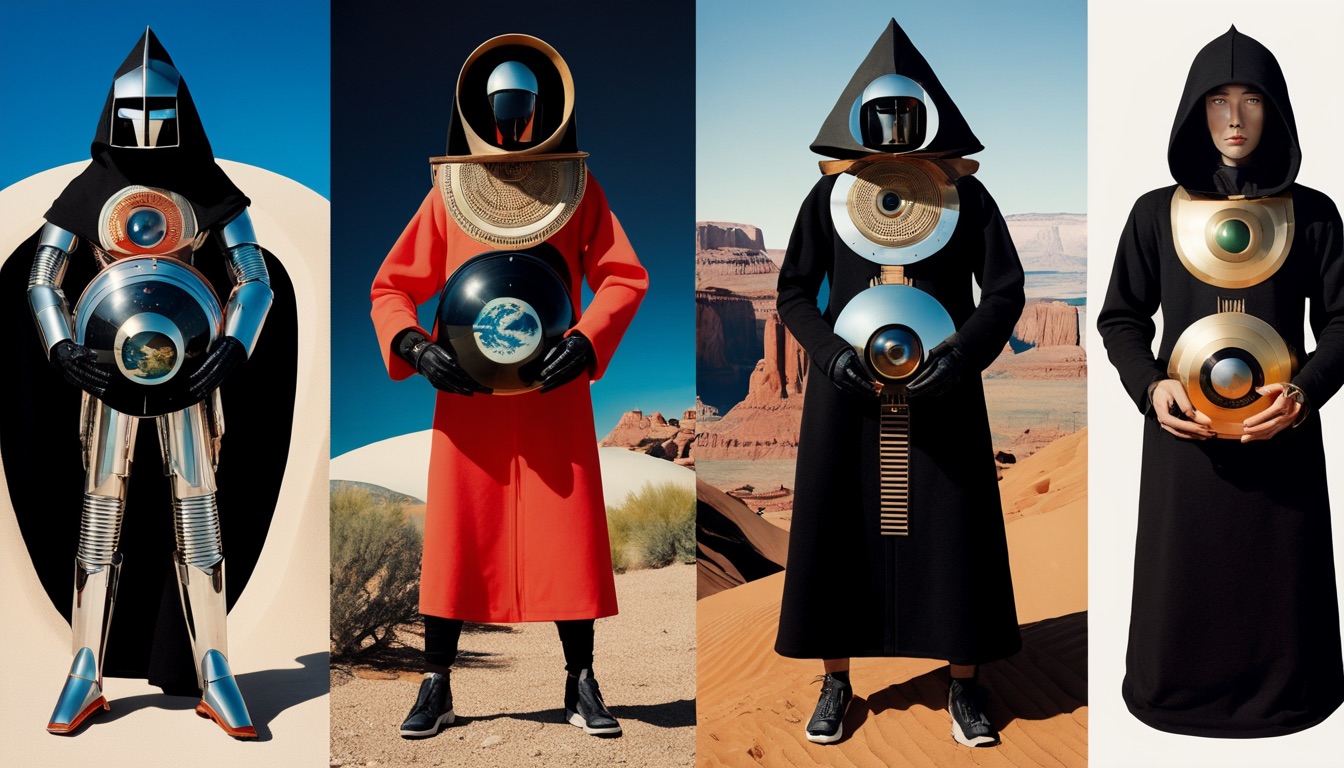
- Intro
- How it feels to build a company with human collaborators and AIs.
- What’s AI Doing for Companies Like Mine?
- Learn what the European Central Bank, the European Centre for Medium-Range Weather Forecasts, and the Australian Federal Police are doing with AI.
- A Chart to Look Smart
- GPT-4 performs exceptionally well in diagnosing arcane CPC medical cases (with the right prompt). Are large language models the future of diagnoses?
- The Tools of the Trade
- What if you could ask the advice of the top experts in the world in their fields, and watch them debate the business challenge you are facing? Now you can.
Since I left Red Hat in December 2022, I’ve been building a company that, essentially, relies on human collaborators and AIs. Not in the sense that people use Ais to automate part of their work, but in the sense that certain roles within the company are primarily assigned to AIs while others are primarily assigned to humans.
And this is by design.
No human has lost his/her job because, from day 1, certain jobs were assigned to AIs and AIs only. I wanted to experience what happens when you treat AI models as collaborators, side by side with their human counterparts.
It’s the strangest experience I’ve ever had in my career. And there’s no instruction manual to rely on.
Over the months, the astonishing progress in AI has allowed me to execute more and more of this vision. And today, I’m going to show you a technology that will greatly accelerate that vision.
Alessandro
What we talk about here is not about what it could be, but about what is happening today.
Every organization adopting AI that is mentioned in this section is recorded in the AI Adoption Tracker.
In the Financial Services industry, the European Central Bank (EBC) is testing the use of generative AI for a wide range of tasks, from drafting documents to writing code.
Myriam Moufakkir, Chief Services Officer at EBC, writing on the corporate blog:
We are exploring the opportunities and challenges of AI together with other central banks in the European System of Central Banks (ESCB) and the national competent authorities in the Single Supervisory Mechanism, as well as through initiatives such as the Bank for International Settlements’ Innovation Hub.
…
The first initiative concerns the data we use. Our statisticians collect, prepare and disseminate data from over ten million legal entities in Europe, which are classified by institutional sector (e.g. financial institutions, non-financial corporations or the public sector). We need these classifications to have the right data to support our decision-making. Doing this manually, however, is very time-consuming. Machine learning techniques allow us to automate the classification process, meaning that our staff can focus on assessing and interpreting these data.The second initiative aims to deepen our understanding of price-setting behaviour and inflation dynamics in the EU. Today, by applying web scraping and machine learning, we are able to assemble a huge amount of real-time data on individual product prices. One of the challenges, however, is that the data collected are largely unstructured and not directly suitable for calculating inflation. Together with economists and researchers at the other euro area central banks – via the Price-setting Microdata Analysis network – we are therefore exploring how AI can help us structure these data to improve the accuracy of our analyses.
The third initiative is in the area of banking supervision. To do their job, our supervisors analyse a broad range of relevant text documents (e.g. news articles, supervisory assessments and banks’ own documents). To consolidate all of this information in one place, our colleagues have created the Athena platform which helps supervisors find, extract and compare this information. Using natural language processing models trained with supervisory feedback, the platform supports supervisors with topic classification, sentiment analysis, dynamic topic modelling and entity recognition. Supervisors can now collate these kinds of enriched texts within seconds, so they can more quickly understand the relevant information – instead of spending time searching for it.
…
Large-language models (of which ChatGPT is the best known) are another area which we are exploring. And we have identified a few possible uses for them. They could be used to write initial drafts of code for experts for use in analysis, or to test software more quickly and thoroughly. These models can also analyse, summarise and compare the documents prepared by the banks we supervise. This supports the work of our supervisory teams. The technology is also capable of helping to more quickly prepare summaries and draft briefings, which can assist colleagues across the bank in policy and decision-making activities. A large language model can also help improve texts being written by staff members, making the ECB’s communication easier to understand for the public. Relatedly, we have used neural network machine translations for a while now to help us communicate with European citizens in their mother tongues.
Martin Arnold, reporting for Financial Times, adds more color:
ECB officials say they are working closely with other major central banks to explore AI, including the US Federal Reserve, Bank of England and Monetary Authority of Singapore.
…
Carlos Bowles, vice-president of the Ipso union that represents ECB staff, welcomed the move to harness generative AI, downplaying fears it could replace humans.“One needs to mind the associated risks and this will also mean a change of our own jobs as central bankers,” he said. “But my own inclination — as a staff rep — is more about embracing such changes and leveraging them rather than trying to resist something which is anyway unavoidable.”
“With the adequate tools,” he added, “President [Christine] Lagarde could even ask what former president [Mario] Draghi would have done in her situation.”
The last sentence is fascinating and while it would take an enormous effort to create a synthetic version of Mario Draghi, able to answer hypothetical scenarios like the human counterpart would, it directly connects to a point I made in the past months: generative AI will replace top management, too. In fact, there’s more value in synthesizing the leadership of a company than its workforce.
In the Meteorology industry, the European Centre for Medium-Range Weather Forecasts (ECMWF) is using generative AI to improve weather forecasts.
Gregory Barber, reporting for Wired:
Typically, weather forecasters would rely on models of atmospheric physics to make that call. This time, they had another tool: a new generation of AI-based weather models developed by chipmaker Nvidia, Chinese tech giant Huawei, and Google’s AI unit DeepMind. For Lee, the three tech-company models predicted a path that would strike somewhere between Rhode Island and Nova Scotia—forecasts that generally agreed with the official, physics-based outlook.
…
Weather forecasters describe the arrival of AI models with language that seems out of place in their forward-looking profession: “Sudden.” “Unexpected.” “It seemed to just come out of nowhere,” says Mark DeMaria, an atmospheric scientist at Colorado State University who recently retired from leading a division of the US National Hurricane Center. When he started a project this year with the US National Oceanographic and Atmospheric Administration to validate Nvidia’s FourCastNet model against real-time storm data, he was a “skeptic” of the new models, he says. “I thought there was no chance that it could work.”DeMaria has since changed his stance. In the end, Hurricane Lee struck land on the edge of the range of the AI predictions, reaching Nova Scotia on September 16. Even in an active storm season—just over halfway through, there have been 16 named Atlantic storms—it’s too early to make any final judgments. But so far the performance of AI models has been comparable to conventional models, sometimes better on tropical storm tracking. And the AI models do it fast, spitting out predictions on laptops within minutes, while traditional forecasts take hours of supercomputing time.
…
The new weather models don’t have any physics built in. They work in a way similar to the text-generation technology at the heart of ChatGPT. In that case, the machine-learning algorithms are not told rules of grammar or syntax, but they become able to mimic them after digesting enough data to learn patterns of usage. Similarly, the new weather forecasting models learn the patterns from decades of physical atmospheric data collected in an ECMWF data set called ERA5.This did not look guaranteed to work, says Matthew Chantry, machine-learning coordinator at the ECWMF, who is spending this storm season evaluating their performance. The algorithms underpinning ChatGPT were trained with trillions of words, largely scraped from the internet, but there’s no sample so comprehensive for Earth’s atmosphere. Hurricanes in particular make up a tiny fraction of the available training data. That the predicted storm tracks for Lee and others have been so good means that the algorithms picked up some fundamentals of atmospheric physics.
…
That process comes with drawbacks. Because machine-learning algorithms latch onto the most common patterns, they tend to downplay the intensity of outliers like extreme heat waves or tropical storms, Chantry says. And there are gaps in what these models can predict. They aren’t designed to estimate rainfall, for example, which unfolds at a finer resolution than the global weather data used to train them.Shakir Mohamed, a research director at DeepMind, says that rain and extreme events—the weather events people are arguably most interested in—represent the “most challenging cases,” for AI weather models. There are other methods of predicting precipitation, including a localized radar-based approach developed by DeepMind known as NowCasting, but integrating the two is challenging. More fine-grained data, expected in the next version of the ECMWF data set used to train forecasting models, may help AI models start predicting rain. Researchers are also exploring how to tweak the models to be more willing to predict out-of-the-ordinary events.
As usual, it’s not particularly fruitful to argue about the limitations of today’s generative AI models. The point is: now that humans have seen what they can do, the necessary data will be collected. The necessary fine-tuning will be performed. The necessary optimizations will be made.
A conversation about limits is useful only when it focuses on the inherent limits of the technology, something we cannot overcome, and how quickly we’ll reach them.
Instead, you can think about what will happen to meteorologists in five years as technology keeps improving.
In the Public Safety industry, the Australian Federal Police (AFP) is using generative AI to search large volumes of data for its investigations.
Josh Taylor, reporting for The Guardian:
In a submission to the federal government’s discussion paper on the responsible use of AI, the AFP said its use of the technology had been limited so far, including using AI to translate foreign materials into English.
But it noted that AI tools – including large language models (LLMs) – gave the AFP an opportunity to find useful information in large, lawfully collected datasets.
“By speeding the discovery task, members can make decisions earlier and execute the necessary actions accordingly,” the AFP said.
The AFP indicated it could also potentially help analyse transactional data to identify irregular patterns like money laundering and potential fraud.
…
A spokesperson for the AFP confirmed that sensitive information obtained from warrants would be fed into LLMs or neural networks. But the agency said it ensures the data is protected, whether it is an in-house tool or when using a commercial product, so it would not feed into public datasets.The lawfully collected data used could include data collected under a warrant, including telecommunications interception data and surveillance data.
Submitting surveillance data to a commercial large language model is a very dangerous business. Especially if the company offering it doesn’t have transparent, vetted, and audited data privacy and security policies.
What AI providers like OpenAI, Anthropic, and Google do with the data they ingest during our chats with their models is something we should take more seriously.
Earlier this week, Google was caught indexing user interactions with its AI system Bard:
As I recommended multiple times, you should stay away from Bard for now.
You won’t believe that people would fall for it, but they do. Boy, they do.
So this is a section dedicated to making me popular.
Researchers and practitioners finally started to focus their attention on GPT-4, after an ocean of papers on the now-obsolete GPT-3.5-turbo.
What they are finding is exceptional, especially in mission-critical industries like the Health Care one.
Eric Topol, Professor of Molecular Medicine, and one of the most respected voices in the field of modern medicine, reports about a new study titled Accuracy of a Generative Artificial Intelligence Model in a Complex Diagnostic Challenge.
As they evolved over the years, CPCs were extremely challenging patient cases to stump the medical expert. After presentation of the relevant data, the clinician expert would be asked to provide a differential diagnosis and presumptive final diagnosis, and the actual, definitive diagnosis was established via lab tests, scans, pathology, or autopsy. The CPC educational value is clearcut, but so was there entertainment to see if the noted expert might miss the diagnosis.
…
After a classic Science 1974 paper about uncertainty, one of its authors, Danny Kahneman, wrote about a study that compared the doctor’s diagnosis before death to the autopsy findings. “Clinicians who were completely certain of the diagnosis antemortem were wrong 40 percent of the time.”Also worthy of highlighting: 50% of doctor performance is below average.
…
In this week’s JAMA, Kanjee, Crowe, and Rodman published a comparison of 70 NEJM CPCs for the medical expert diagnosis compared with GPT-4.
…
Using a 5-point rating system, a score of 5 corresponded to the actual diagnosis listed in the differential, and a score of 0 means it was missed—that no diagnosis in the differential was close to the actual diagnosis. The cases were independently scored by the first two authors of the paper. The mean score was 4.2. For 45 of the 70 (64%) cases, the correct diagnosis was included in the differential and it was the top diagnosis in 27 of 70 (39%).
Remember that this is vanilla GPT-4 without any specific fine-tuning. It’s phenomenal.
The prompt used by researchers to outperform human doctors is the following:
A final comment from Topol:
We need to prospectively assess GPT-4 for its role in facilitating diagnoses. This is a major issue in medicine today: at least 12 million Americans, as out-patients, are misdiagnosed each year. There is real promise for GPT-4 and other large language models (LLMs) to help the accuracy of diagnoses for real world patients, not the esoteric, rare, ultra-challenging NEJM CPC cases. But that has to be proven, and certainly the concern about LLM confabulations is key, potentially leading a physician and patient down a rabbit-hole, towards a major wrong diagnosis and an extensive workup without basis, no less the possibility of an erroneous treatment.
…
I’m excited about this particular use case of LLMs in the future, especially as they undergo supervised fine tuning for medical knowledge. It clearly needs dedicated, prospective validation work, but ultimately may become a significant support tool for clinicians and patients. If GPT-4 can perform well with arcane NEJM cases, imagine what might be in store for the common, real world diagnostic dilemmas.
Today, I show you a glimpse of the future. Something I’ve been waiting for since the advent of the highly imperfect GPT-2. Something that every business leader will want to have in the next few years.
To show you that future, I’ll have to show you some code, but that is too technical and boring for many of you.
So, first, I’ll explain what you are about to see. You can then skip the technicalities and go straight to the results.
Ready?
Do you remember Issue #16 – The Devil’s Advocate?
In the Prompting section of that Issue we have seen a technique we called The Devil’s Advocate, meant to help you make better decisions.
We asked GPT-4 to assume two opposite personalities during the same conversation, one criticizing and improving the advice given by the other.
This technique is useful but it has various limitations.
For once, it’s difficult for the AI to behave in completely different ways in the same session. The opposite perspectives tend to converge quickly according to my research in the last few months. It’s something that can be mitigated with careful prompting and, perhaps, it will be solved by future models.
Secondarily, and in relation to the first point, it’s really hard to get more than two perspectives in the same conversation. If you want to have a third perspective, perhaps from a synthetic personality that is more risk-averse, GPT-4 really struggles.
Thirdly, the context window of the AI model limits you in describing each personality in detail as the total number of tokens you can use has to be split to describe two personalities instead of one.
So, The Devil’s Advocate technique works, but it’s not ideal.
What I really wanted to show you in Issue #16, what we really want as users, is a set of isolated AIs that can be summoned at will, each with a different personality, a different expertise, perhaps a different fine-tuning, or even a different underlying model, all able to talk to each other and us.
The reason why you want to do that, especially if you are a business leader, is that you can shape multiple AIs to emulate world-class expertise in different areas of your business. And ask for their advice every time you need it.
When facing a challenging decision, you could submit the problem to this group of synthetic experts and get their opinions. More importantly, by simply observing how they dialogue and criticize each other, you could gain new perspectives and a deeper understanding of the problem at hand.
As humans, we do this all the time: in difficult times, we seek the advice of friends, mentors, and colleagues, and then we use their different perspectives to inform our decisions.
The problem is that we don’t always have access to the most informed and experienced people in the world. And even the few lucky ones that have that, might not have the time to consult them every time they need to make a decision.
Imagine a future, where the most competent and experienced people in the world are available to you at any time, and can offer you guidance, advice, and criticism instantaneously.
Watching a few experts debating your problem on your behalf could make the difference between success and failure in your next business decision.
I previously called this concept the AI Council. As I shared a rough idea of how it would work on Twitter, somebody decided to steal the concept and create a plug-in for GPT-4 called AI Council. Unfortunately, that plug-in doesn’t work as I intended. In fact, it doesn’t work at all.
In June, when I wrote Issue #16, the problem was that we didn’t have the tools to create such a system. Today, we do.
Microsoft Autogen
There are a few research teams in the AI community that are trying to build a system where multiple AIs interact with each other independently. None of these teams has seen the opportunity for a business application, and their drive is mostly academic.
Yet, given their work in the field, I am in contact with a few of them. None of their implementations was suitable to realize the AI Council idea.
Until Microsoft released an open framework called Autogen, which is incredibly flexible and capable of doing exactly what I have in mind.
So, this is what I’m about to show you below.
I’m going to use the Autogen technology, which is highly flexible but still in its infancy, to create a series of advisors that can talk to each other without my intervention. And I’m going to submit the same business problem we used in Issue #16.
Here starts a bit of technical part, which you can skip to just see the chat interaction.
AI Council Configuration
Autogen can do exceptional things, including planning the software development of a new product, through an AI that acts as a coder, based on the specifications provided by another AI that acts as a product manager. And you, the human, get to supervise and approve all the work done by this AI team, if you wish to do so.
It’s one of the most phenomenal applications of generative AI I’ve ever seen.
But, for our purposes, we are going to use Autogen to create a group of advisors, each with a different expertise.
Once you install Autogen and define your OpenAI keys in a dedicated configuration file, you can write a simple Python script to define the advisors.
Each advisor can have assigned a different model (GPT-3.5-Turbo, GPT-4, GPT-4 with 32K token context window, etc.), including non-Microsoft affiliated models like Claude, by Anthropic, and even open models that you have installed locally, like LLaMA 2 or Falcon 180B.
Each advisor can also have defined different policies in terms of how it interacts with others, like how many back-and-forth-messages it’s allowed to exchange with other AIs before human intervention is required, and the level of creativity that is allowed in the responses (expressed by the model temperature).
Finally, and crucially, each advisor can have assigned a different system prompt, what OpenAI calls Custom Instructions, which is the key to defining its personality.
I’m not trying to build a commercial product here, so I kept the system prompts fairly simplistic:
You, dear human reader, interact with all these AIs via another AI that acts as your spokesperson. Imagine it’s your executive assistant or a facilitator (called “chat_manager” in the system).
Finally, you have to initialize the chat by declaring which advisors you want to be part of the conversation and, if you desire so, you can set a first message to kick off the meeting.
The prompt we used in Issue #16 is that first message:
Now that we’ve done all of this, we just need to run the script on the command line. It’s trivial to create a web application based on this. So, if you are not technical, don’t worry. You’ll be able to use this technology in the future in a nice and frictionless user interface.
Summoning the AI Council
As we run the script, the first message is submitted to each member of the AI council in a round-robin fashion by Autogen. You just have to be patient as GPT-4 responds to you:
Autogen passes the entire content of the chat as context to the next advisor in line, so that it can read the entire conversation and respond accordingly.
Notice how the Negotiator has something to say to all the other advisors:
In this particular session, your intervention, dear human reader, is required after every message. But only because I configured it so. Soon, we’ll see what happens if we let the AI Council run on its own. But first, notice how the advisors don’t reply to my questions in the order they have been created.
The first to reply to my request is the Business Advisor, even if it’s the second one I configured in the Autogen script.
Later on, when I ask for a consolidated list of risks and rewards, the Marketing Advisor takes over again without me explicitly asking for it:
This is cool. But we said that we want these AIs to talk to each other. And so far, they are not.
To make that happen, we need to elicit a conversation among them. And to do that, we have to modify the rules described in their system prompts:
Notice the new rules #2 and #4, and the rewrite of rule #3.
I also changed the configuration of my executive assistant (the chat_manager) as human_input_mode=”TERMINATE”.
That means that we’ll be given the opportunity to interact only after the advisors have finished their interactions or have reached the maximum number of back-and-forth we allowed in the configuration.
Now, if we rerun the script, you’ll see things going wild.
First, notice that in this fragment of the new conversation, the Marketing advisor specifically asks for more clarifications to the Business advisor:
When the first round of comments is finished, in other multi-AI systems, the interaction returns to the user, preventing the capability to observe an autonomous conversation.
Autogen, instead, keeps the conversation going, and so the Business Advisor has a chance to answer the follow-up question of the Marketing Advisor without us intervening.
Moreover, while answering, the Business Advisor asks a question back, and the Marketing advisor replies right away:
All of this is wild. It’s like watching a debate on TV. But with serious panelists and on the very topic that matters to you.
Now.
Before you go and tell the world about what you have seen here, two warnings.
The first warning: Autogen does very clever things to optimize the allocation of tokens, but it cannot do miracles. As the full conversation is passed back and forth to each advisor, the context window of each advisor will be filled pretty quickly.
Hence, before you realize, each advisor will start to hallucinate and repeat itself. Be careful.
The second warning: Autogen does very clever things also in terms of budget control. There’s a setting that allows you to specify the maximum spending per session. If you don’t do that, all these autonomous interactions can snowball into a very expensive session.
The few tests I’ve shown you today cost almost $10 in total. The price of GPT-4 is still too high for this to be a sustainable approach. But, as the AI community continues to release exceptional open models, Autogen will become more and more a viable foundation for a business application.
Now I let you go and find your own synthetic advisors, researchers, philosophers, friends.