October 28, 2023
Splendid Edition
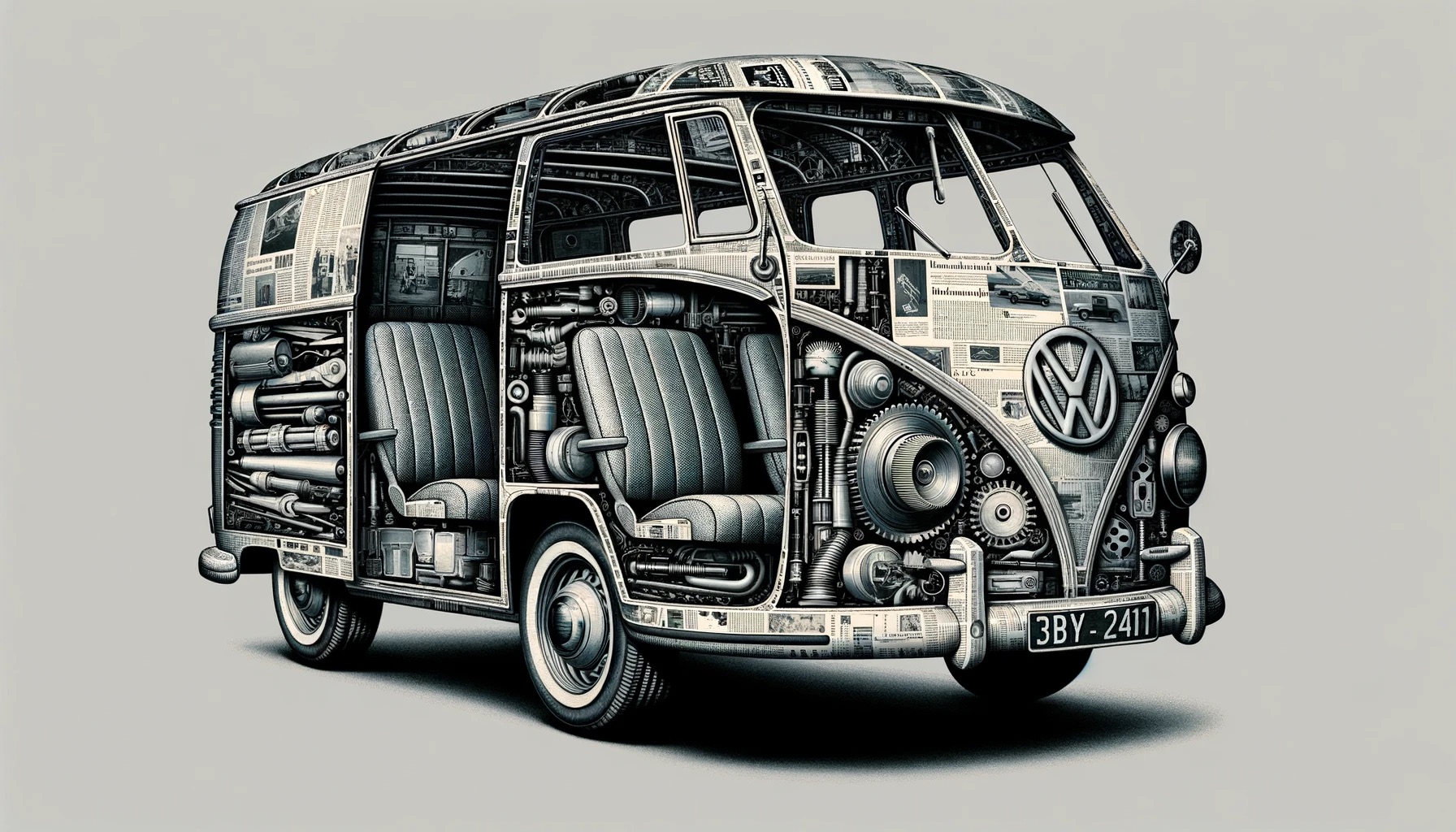
In This Issue
- What’s AI Doing for Companies Like Mine?
- Learn what Deliveroo, Moody’s, and Amazon are doing with AI.
- A Chart to Look Smart
- A creative application of generative AI produces accurate simulations of disease progression with limited data.
- The Tools of the Trade
- A local ChatGPT that, finally, can be used to chat with multiple documents (yes, Excel spreadsheets, too).
You need a paid membership to read this content.