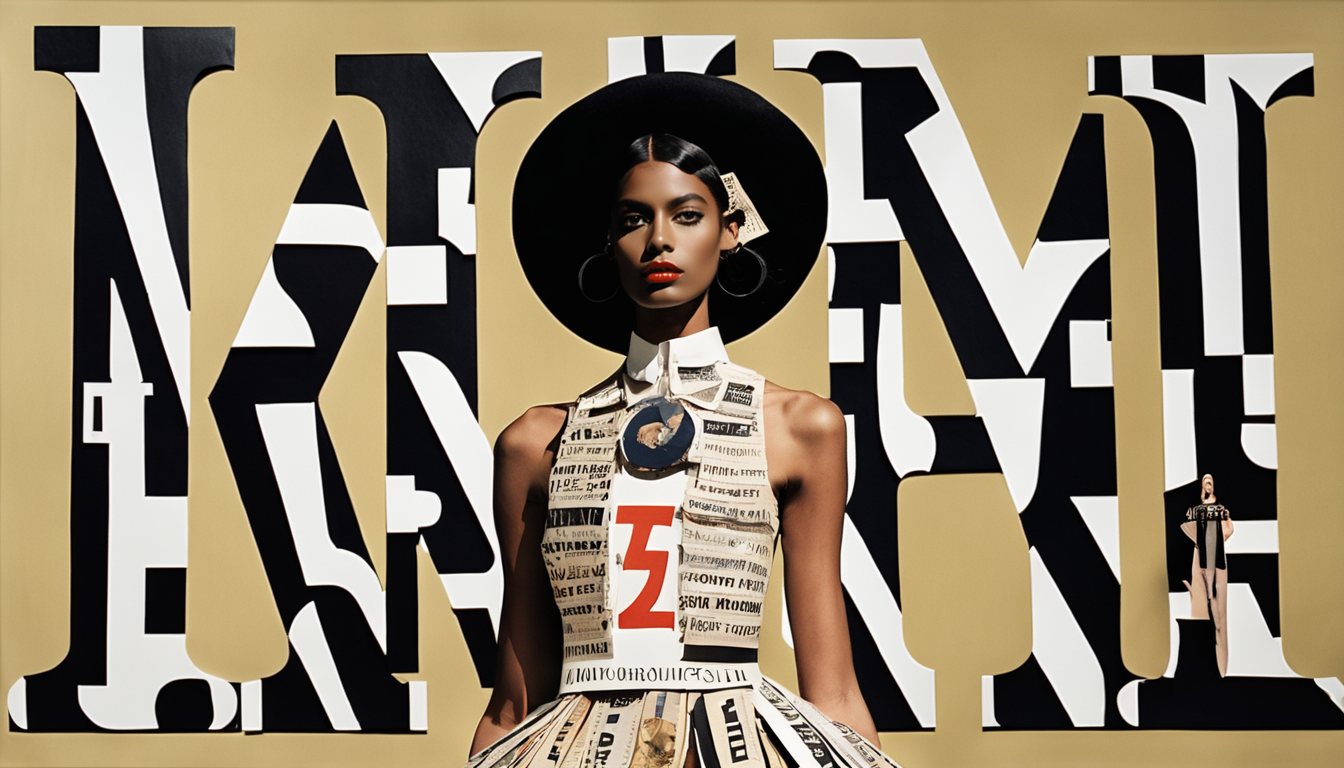
- Intro
- Generative AI is influencing the way we behave. As individuals, as workers, as companies and, eventually, as a society.
- What Caught My Attention This Week
- If fashion is a language, can a large language model manipulate it as it does with English?
- Will traffic police exist in a future full of self-driving cars and AI-powered traffic lights?
- Stack Overflow reduced its headcount by 28%. Who will be next?
- The Way We Work Now
- Australian schools have capitulated to artificial intelligence.
Everybody enjoys large language models, but nobody wants to feed them for free anymore.
One by one, every content platform on the Internet is shutting its doors. Either by shutting down RSS access (e.g.: X/Twitter), by asking for unsustainable sums to access their APIs (e.g.: Reddit), or by blocking access to web scraping (e.g., BBC).
This is not just something we read in the news. For 20+ years, I’ve used the RSS protocol to absorb and research enormous quantities of data, and I can confirm that it’s getting harder and harder to access information.
Where the triage of that information used to take seconds, it now takes hours, and it’s becoming unsustainable.
Everybody loses in this.
A core tenet that made the Internet the exceptional place it is today, open access, is crumbling either out of greed or out of fear. The two great emotions that move the world.
The ones moved by greed feel entitled to use an AI model that has been trained on a dataset contributed by the whole planet by paying only $20 / month or less, all the way to zero. Yet, they want to be compensated for contributing their content to make that very model even better.
The irony is that, in some cases, the content of these platforms is not theirs. It has been generated by the people that use their platform. Yet, no user will ever see a percentage of the profits made by the platform for paid access to their APIs. And no platform shutting its doors to AI providers has consulted its users before doing so.
While it’s true that a content platform invests in cloud infrastructure to host the user content, it’s also true that it profits from it via advertising, user profiling, and other means. And what AI providers have amply demonstrated, what’s truly valuable is the content, not the platform.
Putting aside what’s written in terms of service agreements, there’s a remarkable sense of entitlement in shaping your business around the belief that just because you host content, you own it.
Imagine Amazon Web Services having that attitude about the content hosted on its servers by its customers worldwide.
This sense of entitlement is the same that now emboldens content platforms to train their AI models on our content without asking for permission in a clear and comprehensible way (it’s in the ToS).
Here’s the new X ToS:
Alex Ivanovs, writing on Stackdiary:
based on these AI-assigned definitions in the updated terms, your access to certain content might be limited, or even cut off. You might not see certain tweets or hashtags. You might find it harder to get your own content seen by a broader audience. The idea isn’t entirely new; we’ve heard stories of shadow banning on Twitter before. But the automation and AI involvement are making it more sophisticated and all-encompassing.
This shift isn’t just about Twitter. Imagine this methodology expanding across the entire internet. Imagine if your “definitions,” determined by AI, controlled not only what you could see on Twitter but also what search results appeared for you on Google, or which news stories showed up in your Facebook feed.
It’s not that the internet has fewer pathways or offramps; it’s just that you might not see them all. They are hidden or revealed based on your AI-defined identity. So while the internet remains vast and expansive, your individual experience of it could become very narrow, indeed.
And speaking of Amazon, they are on a very dangerous slippery slope.
Usually, that sense of entitlement bites back as active contributors migrate elsewhere as soon as a viable alternative appears, and the offending platform is left with a bunch of passive consumers who won’t generate anything of substance, pushing the platform into an irreversible spiral of death.
Perhaps, not this time. Perhaps, the LLMs we already have today will be used to generate synthetic content good enough, for long enough, that the platforms will survive even without a human driving force.
Even if so, eventually, the human creators that have been pushed away will find a new home and that home will represent a competitor to confront with.
The ones moved by fear feel entitled to use an AI model that has been trained on a dataset contributed by the whole planet to generate novels, photographs, drawings, paintings, book plots, film scripts, etc. All things they profit from: directly, with monetary compensation, or indirectly, via status gain. Yet, these people don’t want their content to be copied and used to compete against them.
An example, as reported by Morgan Sung for Techcrunch:
October is a bacchanal of fan fiction, from romantic one-shots about unconventional character pairings to delicious smut that’ll make you reconsider your own sense of morality — all inspired by the month’s countless themed writing challenges. It’s an especially busy time for the fan fiction site Archive of Our Own (AO3).
But this year’s monthlong prompt festival may seem quieter to the casual AO3 reader, with popular writers’ work seemingly wiped from the site altogether. In most cases, the stories still exist, but they aren’t publicly viewable anymore.
In an effort to prevent their writing from being scraped and used to train AI models, many AO3 writers are locking their work, restricting it to readers who have registered AO3 accounts. Though it may curb bot commenters, it also limits traffic from guest users, which can be a blow for newer and less popular writers.
…
At the time of reporting, over 966,000 of the roughly 11.7 million works on AO3 were accessible only for registered users. It’s just a fraction of AO3’s vast library of content, but it’s worth noting that many authors are only locking new work, since existing fics were likely already scraped.
…
Some readers took to Tumblr and Twitter to ask their favorite fan fiction authors if they had taken down their writing. One asked AO3 writer takearisk to unrestrict their work so they could read it on Tumblr, where many use RSS feeds to keep up with new chapters.“thanks so much for reading!! but no,” takearisk responded on Tumblr last week. “i had some ai bot comments a few months back that really freaked me out and i also found one of my older marvel fics posted to another site without my permission. it’s something that i never wanted to have to do, but i put way too much effort into my fics to be okay with them being stolen. so i am going to keep my account locked for the foreseeable future.”
…
Some users speculate that data scraping tools automatically leave comments to “make their browsing traffic look legitimate,” according to one Tumblr user. AO3 users also speculate that the comments are being used to test AO3’s spam detection filters, or that they’re an attempt to encourage writers to keep their fics public and scrapable. AO3 authors have been accused of posting AI-generated fiction, or have expressed concern that the positive comments they leave on other writers’ fics will be misconstrued as AI spam.
Of course, these acts of plagiarism do not lead to the publication of an unauthorized book that becomes a New York Times bestseller. Yet, seeing your work shamelessly copied and published without permission on another website where 12 people will read it is enough to justify an asymmetric use of AI.
More than 20 years ago, I was publishing another media project called virtualization.info. Unquestionably, that project propelled my career internationally and let my trajectory until this very moment, as I type this newsletter for you.
At that time, somebody had the brilliant idea of copying both the domain name and the content with a much more rudimentary technology than today’s AI.
As irritating as it was, it never impacted the economic success of the original project and never fooled more than a handful of readers out of millions I could count on. I never thought of restricting access to the content because that was not the business model I felt was right for that project.
What would have happened if I had kept virtualization.info behind a registration wall?
It’s possible that virtualization.info wouldn’t have reached millions of readers becoming the reference point for the virtualization industry.
It’s possible that my career would have taken a much different, less successful trajectory.
What does all of this have to do with Synthetic Work?
This:
Generative AI is not just another tech that will make us do things a little bit differently, a little bit better. Generative AI is influencing the way we behave. As individuals, as workers, as companies and, eventually, as a society.
It’s forcing us to rethink what is “fair use”, what is a “product”, what it means to say “I made it”, or what it means to say “I own it”. And all of this, in turn, forces us to rethink our competitive strategies and our business models.
This is not business as usual.
Alessandro
I rarely talk about startups focused on AI. For most of them, there’s too much hand-waving, unhealthy business propositions, or thin layers of unsustainable differentiation to justify a recommendation. I mention the few ones that I feel confident about and I use on a daily basis in the Splendid Edition of Synthetic Work.
This week, I’ll make an exception for Aiuta, a startup building a synthetic fashion assistant, which is doing remarkable work in fine-tuning open access large language models.
Their approach to fashion is intriguing:
Our fundamental belief is that fashion is also a language. Following this perspective, we created a technology that is able to understand whether a certain outfit looks fashionable or not and come up with more matching options.
Why is fashion a language? Just as words form sentences to convey meaning, fashion items combine to create outfits that communicate a particular style or message. In this context, fashion can have its own vocabulary (clothing items) and item-arrangement grammar within an outfit and combination rules. Just like in human language, there’s room for mistakes: we can speak a language poorly and we can dress badly. The rules of fashion, though, are much less tangible than the grammar of language. People do not often agree on what is “right” to wear, yet a certain code exists — wearing a meaningless outfit can be just as disappointing as saying a meaningless phrase. Also, there are ways of dressing that are almost universally scorned, such as the notorious “socks and sandals” combination; fashion icons and events continually try to corral the public towards a certain shared ideal of look.
Viewing fashion as a language allows us to leverage computational approaches for analyzing and generating fashion.
Once we consider garments as visual entities, using pictures and photos to build a representation we can learn to understand the “visual language” of outfits. This knowledge can then be used to recognize patterns, style preferences, and trends in order to recommend personalized outfits, generate new fashion combinations, or even predict future fashion directions. We decided to call our technology FashionGPT — Fashion Garment Pretrained Transformer.
To put it simply, we see an outfit as a sentence. A “bad” outfit stands for an erroneous or inexact sentence, while a “good” one represents a meaningful and consistent one.
Just as a sentence is composed of different words, an outfit is composed of individual garments. When processing natural language, algorithms use various techniques to split text into words and subwords. Similarly, in the visual domain, outfits and collages can be split into image patches, to allow a finer-grained analysis. Different garments characterized by the same shape and color may be considered as synonyms.
Based on these strong similarities, we reasoned that some sort of LLM-like approach could also do the trick here. Our concept is simple yet striking: fashion language modeling can provide us with stable representations for garments and outfits, to be used for both scoring and recommendation.
…
In the past years, several research teams investigated the use of transformers and language models for analyzing outfit compatibility through image captioning or swin transformer networks, relying on pre-trained models with no human feedback. An “AI fashioner” based on (pre-transformers) deep learning and computer vision techniques has also been proposed, as well as an image-based fashion recommender based on user interests that, however, models the social aspect of the problem rather than the visual language of outfits. Interestingly, some researchers have looked at “personalized” recommendations based on physical attributes, rather than outfit compatibility.Aiuta’s approach, on the contrary, leverages state-of-the-art transformer models coupled with human input and savvy strategies for augmenting the training data and generating negative examples of outfits.
Long story short, their FashionGPT model is at the top of the HuggingFace LLM Leaderboard.
Why is this so interesting?
The first reason is, obviously, the implications of this technology in terms of occupation within the Fashion and Retail industries. Personal shoppers, stylists, and even fashion designers are all jobs that will be impacted if fashion can be turned into a language and that language can be successfully manipulated by a machine.
So far, LLMs have been very successful with natural languages, code, and music. Why not this?
The second reason, even more interesting and with broader implications, is: what else can we turn into a language?
Do you remember this?
If you have no idea what you are looking at, let me explain: this is the famous Piazza Venezia at the very center of Rome, Italy. And this man is a particular type of traffic policeman colloquially known as “Pizzardone” (literally, “pizza box”).
Before moving to London, I spent most of my life walking or driving through this square, and I’m old enough to have seen this traffic policeman in action.
He’d stand on this platform, which emerges from the street level as necessary, directing traffic with his arms and a whistle during days of particular traffic chaos.
In my personal experience, this figure has not once optimized traffic flows. On the contrary, he has made it even more chaotic than ever. Yet, he’s a beloved icon of the city, remembered with nostalgia by all Romans that probably wanted to see him dead at some point in their lives.
If you want to see him in action, a movie from the 60s, called “Il Vigile”, with the ultrafamous Italian comic actor Alberto Sordi will show you the profession in all its glory:
Traffic policemen still exist today, but nobody directs traffic in that manner anymore.
On the contrary, the advent of self-driving cars and the use of AI to optimize traffic flows will make this profession obsolete in the next few years.
Paresh Dave, reporting for Wired:
Each time a driver in Seattle meets a red light, they wait about 20 seconds on average before it turns green again, according to vehicle and smartphone data collected by analytics company Inrix. The delays cause annoyance and expel in Seattle alone an estimated 1,000 metric tons or more of carbon dioxide into the atmosphere each day. With a little help from new Google AI software, the toll on both the environment and drivers is beginning to drop significantly.
Seattle is among a dozen cities across four continents, including Jakarta, Rio de Janeiro, and Hamburg, optimizing some traffic signals based on insights from driving data from Google Maps, aiming to reduce emissions from idling vehicles. The project analyzes data from Maps users using AI algorithms and has initially led to timing tweaks at 70 intersections. By Google’s preliminary accounting of traffic before and after adjustments tested last year and this year, its AI-powered recommendations for timing out the busy lights cut as many as 30 percent of stops and 10 percent of emissions for 30 million cars a month.
…
The company is expanding to India and Indonesia the fuel-efficient routing feature in Maps, which directs drivers onto roads with less traffic or uphill driving, and it is introducing flight-routing suggestions to air traffic controllers for Belgium, the Netherlands, Luxembourg, and northwest Germany to reduce climate-warming contrails.
…
Traffic officers in Kolkata have made tweaks suggested by Green Light at 13 intersections over the past year, leaving commuters pleased, according to a statement provided by Google from Rupesh Kumar, the Indian city’s joint commissioner of police. “Green Light has become an essential component,” says Kumar, who didn’t respond to a request for comment on Monday from WIRED.In other cases, Green Light provides reassurance, not revolution. For authorities at Transport for Greater Manchester in the UK, many of Green Light’s recommendations are not helpful because they don’t take into account prioritization of buses, pedestrians, and certain thoroughfares, says David Atkin, a manager at the agency. But Google’s project can provide confirmation that its signal network is working well, he says.
…
Smarter traffic lights long have been many drivers’ dream. In reality, the cost of technology upgrades, coordination challenges within and between governments, and a limited supply of city traffic engineers have forced drivers to keep hitting the brakes despite a number of solutions available for purchase. Google’s effort is gaining momentum with cities because it’s free and relatively simple, and draws upon the company’s unrivaled cache of traffic data, collected when people use Maps, the world’s most popular navigation app.
…
Rothenberg says Google has prioritized supporting larger cities who employ traffic engineers and can remotely control traffic signals, while also spreading out globally to prove the technology works well in a variety of conditions—suggesting it could, if widely adopted, make a big dent in global emissions.
Traffic policemen out there: reskill to become traffic engineers ASAP. As we said last week, “While we wait for the utopia of a world where nobody has to walk thanks to the cars, everybody might be asked to become a car mechanic.”
The article provides extensive details about the implementation and it’s worth reading in full.
An important question remains unanswered: why is Google giving this system away for free?
In Issue #23 – One day, studying history will be as gripping as watching a horror movie, we saw how the content platform Stack Overflow saw a traffic decline of 40% year after year, likely due to the advent of Copilot and ChatGTP.
Everybody is looking at what will happen to the website as a canary in the coal mine for the future of many other platforms. And what’s happening is not good.
Quoting from the official announcement:
This year we took many steps to spend less. Changes have been pursued through the lens of minimizing impact to the lives of Stackers. Unfortunately, those changes were not enough and we have made the extremely difficult decision to reduce the company’s headcount by approximately 28%.
…
As we finish this fiscal year and move into the next, we are focused on investing in our product. As such, we are significantly reducing the size of our go-to-market organization while we do so. Supporting teams and other teams across the organization are impacted as well. As I mentioned, our focus for this fiscal year and into the next is profitability and that, along with macroeconomic pressures led to today’s changes. As we refine our focus, priorities, and strategy it’s to better meet the demands of our users, customers, and partners as part of this commitment to product innovation and the continued momentum of OverflowAI both for Stack Overflow for Teams and on our public platform.
As a reminder, Stack Overflow reacted too slowly to the advent of LLMs and built the OverflowAI too late to retain its readership.
Perhaps, this was inevitable. Perhaps, reacting to the early signs of a trend with a healthy paranoia would have saved the jobs of many people.
Are you reacting to the early signs of a trend with a healthy paranoia?
This is the material that will be greatly expanded in the Splendid Edition of the newsletter.
Australian schools have capitulated to artificial intelligence.
Caitlin Cassidy, reporting for The Guardian:
Artificial intelligence including ChatGPT will be allowed in all Australian schools from 2024 after education ministers formally backed a national framework guiding the use of the new technology.
The framework, revised by the national AI taskforce, was unanimously adopted at an education ministers meeting on Thursday. It will be released in the coming weeks.
…
Every state and territory excluding South Australia moved to temporarily restrict ChatGPT in public schools as concerns mounted about privacy and plagiarism.But in a communique released on Friday morning, ministers confirmed that state and territories and non-government schooling sectors would work with their own education systems to implement the framework from term 1 next year.
The adoption includes a $1m investment to Education Services Australia – a not-for-profit educational technology company owned by federal, state and territory education departments – to establish “product expectations” of generative AI technology.
…
The body has been liaising with education product vendors since the release of ChatGPT and estimated 90% would move AI into their existing technology within the coming years.
And just like that, in a mere year, the job of the student and the job of the teacher have changed forever.
This week’s Splendid Edition is titled Talk to me.
In it:
- Intro
- Synthetic Work’s AI Adoption Tracker now documents 100 early adopters and use cases around the world
- What’s AI Doing for Companies Like Mine?
- Learn what US Space Force, Accenture, and Spotify are doing with AI.
- A Chart to Look Smart
- The Federal Reserve Bank of St. Louis is exploring the use of generative AI to forecast inflation. The results are surprising.
- What Can AI Do for Me?
- My new AP Workflow 5.0 is ready to generate images at scale for industrial applications
- The Tools of the Trade
- Is the new Descript voice cloning service ready for prime time?