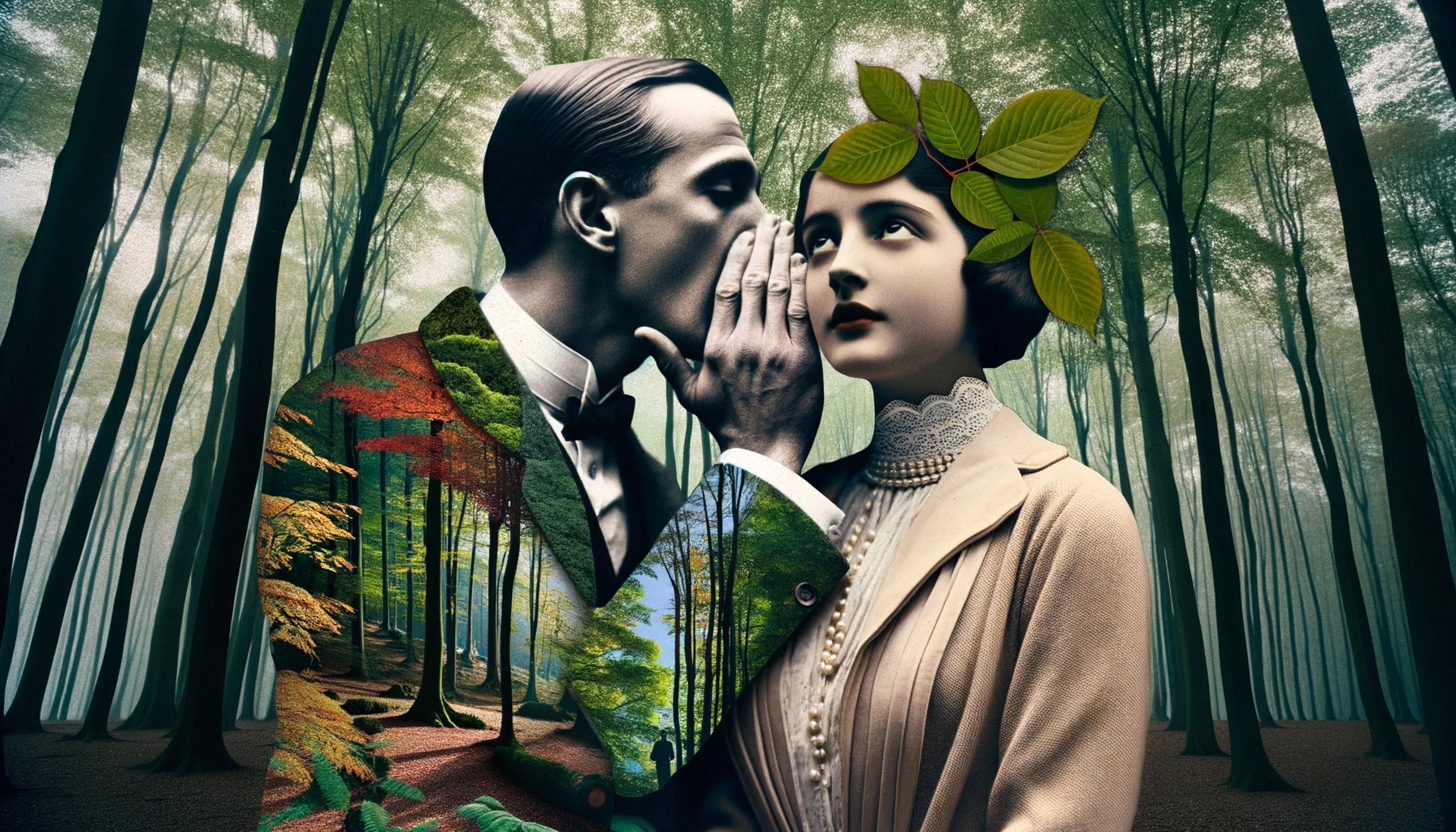
- Intro
- Synthetic Work’s AI Adoption Tracker now documents 100 early adopters and use cases around the world
- What’s AI Doing for Companies Like Mine?
- Learn what US Space Force, Accenture, and Spotify are doing with AI.
- A Chart to Look Smart
- The Federal Reserve Bank of St. Louis is exploring the use of generative AI to forecast inflation. The results are surprising.
- What Can AI Do for Me?
- My new AP Workflow 5.0 is ready to generate images at scale for industrial applications
- The Tools of the Trade
- Is the new Descript voice cloning service ready for prime time?
100! A hundred early adopters across the world are now listed in Synthetic Work’s AI Adoption Tracker.
Of course, there are many more early adopters than a hundred all around the world. But these are confirmed, verifiable, and researchable use cases. Not the handwaving of overexcited (and not necessarily honest) technology vendors.
A tremendous research tool I wish I had when I was an analyst for Gartner a life ago.
I expect to give many presentations to customers about these 100 adopters, as more and more organizations look for guidance and inspiration to adopt artificial intelligence. I’ll start in Italy, this December, where I’m invited by a company in the Professional Services industry to kick off their internal AI hackathon.
To the next 100.
Alessandro